1. Introduction: Where Endurance Meets Intelligence
The world of ultramarathon running is one of extremes. It’s a realm where athletes push the boundaries of human physical and mental endurance, traversing distances that dwarf the traditional marathon, often across challenging terrains and in unpredictable conditions. Preparing for such feats demands meticulous planning and adaptation, increasingly turning athletes towards advanced methods, including AI ultramarathon training strategies. Simultaneously, we live in an era defined by data and artificial intelligence (AI), technologies that are revolutionizing industries by extracting insights and enabling capabilities previously confined to science fiction.
What happens when these two worlds collide? When the raw determination of the ultramarathoner meets the analytical power of Artificial Intelligence? The result is a fascinating landscape where technology is shaping performance, offering potential to optimize training, enhance health, and perhaps redefine human limits through smarter preparation.
This pillar post delves deep into the burgeoning relationship between Artificial Intelligence and the ultramarathon sphere. We will explore how intelligent algorithms and data-driven insights, core components of modern endurance sports AI applications, are transforming the sport.
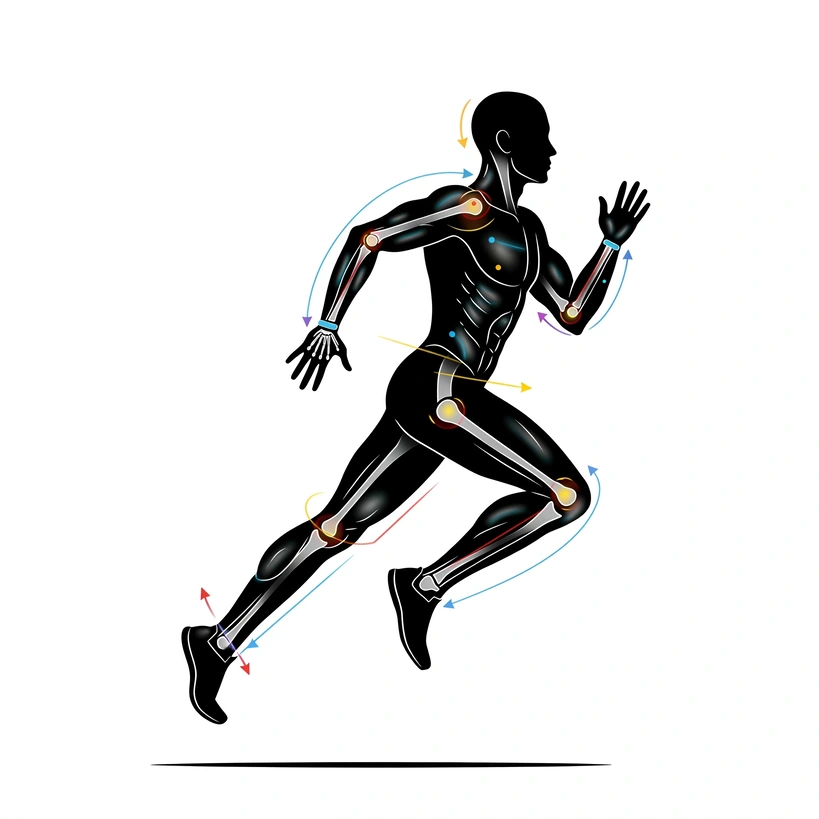
1.1. The Allure and Challenge of Ultramarathons
To understand AI’s impact, we must appreciate the unique nature of ultramarathons – any footrace longer than 42.195km. This includes 50km, 50 miles, 100km, 100 miles, and even time-based or multi-day events. Often held on rugged trails with significant elevation changes, they demand specific adaptations beyond standard marathon prep. The culture emphasizes endurance, mental resilience, and the personal challenge, necessitating highly adaptable training – a prime area where AI ultramarathon training can provide immense value.
1.2. The Power of Data in Modern Sport
Sports have undergone a data revolution. Objective measurements increasingly supplement traditional wisdom. This data explosion, particularly rich in endurance sports, provides the raw fuel for Artificial Intelligence ultramarathon applications designed to optimize every aspect of preparation, turning raw numbers into actionable training insights.
1.3. The Rise of Artificial Intelligence in Sports Science
Within this technological wave, Artificial Intelligence (AI) is rapidly becoming a game-changer in sports science, used for performance analysis, rehabilitation, and strategy optimization. Its application extends significantly into the demanding world of ultra-distance running, particularly through AI ultramarathon training optimization. Beyond just training plans, endurance sports AI applications now touch upon AI injury prediction for runners, personalized nutrition, and even tools acting as an AI running coach for ultras, offering guidance previously accessible only to a few.
1.4. Thesis Statement: The Scope of This Exploration
This pillar post provides a comprehensive exploration of the profound impact of Artificial Intelligence on the ultramarathon world. We will delve into how AI is revolutionizing ultramarathon training, enhancing performance analysis, offering new frontiers in injury prediction, personalizing fueling strategies, and reshaping the athlete’s journey from preparation to recovery. We aim to be the definitive guide on the synergy between AI and ultramarathon endeavors.
1.5. Roadmap: What to Expect in This Post
To guide you through this extensive exploration, this post is structured into key sections. We will navigate the landscape covering AI fundamentals, its deep dive into training methodologies, nutrition, injury prevention, race day potential, recovery optimization, the broader ecosystem impact, ethical considerations, practical examples, and the future outlook for AI in ultramarathon running. Join us as we embark on this journey.
- Part 2: Understanding the Fundamentals: Laying the groundwork by defining ultramarathons and key AI concepts relevant to the sport.
- Part 3: The Training Revolution: Detailing how AI is reshaping training preparation, from data collection to personalized plans and performance prediction.
- Part 4: Intelligent Fueling: Exploring AI’s role in optimizing nutrition and hydration strategies for extreme endurance.
- Part 5: Combating Injuries: Investigating how AI can predict injury risks and aid in proactive management and recovery.
- Part 6: Race Day Intelligence: Discussing the potential (and current limitations) of AI in guiding real-time race strategy and pacing.
- Part 7: The Art of Recovery: Examining how AI helps monitor and optimize the crucial process of recovery.
- Part 8: Beyond the Athlete: Looking at AI’s potential impact on the broader ultramarathon ecosystem, including communities, organizers, and gear.
- Part 9: Ethical Dimensions, Challenges & Future Perspectives: Addressing the critical ethical questions, limitations, and future trajectory of AI in the sport.
- Part 10: Case Studies & Practical Applications: Showcasing examples of AI in action within the endurance community.
- Part 11: Conclusion: Summarizing the key takeaways and offering final thoughts on the symbiotic future of human and machine endurance.
Join us as we embark on this journey into the heart of the AI revolution in ultramarathon running.
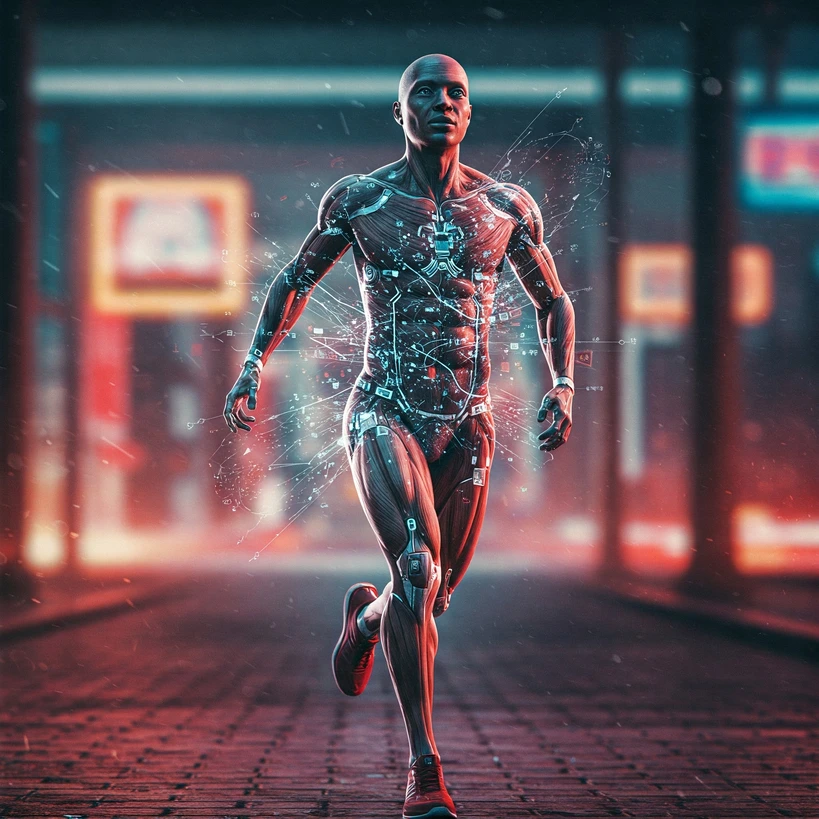
2. Understanding the Fundamentals: Ultramarathons & AI
Before diving into the specific applications, it’s essential to establish a clear understanding of both ultramarathon running and the core concepts of artificial intelligence that are relevant to this discussion.
2.1. The World of Ultramarathon Running
As established, ultramarathons represent a significant step up in distance and often complexity compared to marathons. Let’s break down the key elements further.
2.1.1. Defining the Ultra: Distance, Terrain, Time
- Distance: The minimum threshold is anything over 42.195km (26.2 miles). Common race distances include 50km, 50 miles, 100km, and 100 miles, but events can extend to 200 miles or even further.
- Terrain: While some ultras take place on roads or tracks, many are trail races. This means navigating varied surfaces like dirt, rock, mud, sand, and potentially snow. Significant elevation gain and loss (“vert”) are hallmarks of many trail ultras, requiring specific strength and technical skills for climbing and descending.
- Time: Besides fixed-distance races, time-based ultras (e.g., 6, 12, 24, 48 hours, or multi-day events like 6-day races) challenge athletes to cover the maximum possible distance within the given timeframe. These often take place on shorter, looped courses.
2.1.2. Key Factors for Success
Success in an ultramarathon is a complex interplay of numerous factors:
- Physical Endurance: The ability to sustain effort for extremely long durations is paramount. This involves highly developed aerobic capacity, muscular endurance, and metabolic efficiency.
- Pacing Strategy: Unlike shorter races where athletes might push near their limit, ultras demand conservative pacing, especially early on, to conserve energy and avoid “bonking” (hitting the wall due to glycogen depletion).
- Nutrition and Hydration: Consuming adequate calories, fluids, and electrolytes over many hours is critical and notoriously difficult. Gastrointestinal distress is a common race-ender.
- Mental Fortitude: Ultramarathons are as much a mental battle as a physical one. Athletes must cope with pain, fatigue, monotony, self-doubt, and often, extreme environmental conditions. Resilience and problem-solving skills are essential.
- Gear and Logistics: Proper equipment (shoes, pack, clothing layers, headlamp) suited to the distance, terrain, and expected weather is crucial. Managing logistics, including aid stations and potentially support crews, is also vital.
- Environmental Adaptation: Dealing with heat, cold, wind, rain, altitude, and darkness requires preparation and adaptability.
- Recovery: The ability to recover between demanding training sessions and after races is key to long-term progress and injury prevention.
2.1.3. The Diverse Ultramarathoner Profile
The ultramarathon community is diverse. It includes:
- Elite Athletes: Professionals competing for wins and course records.
- Competitive Age-Groupers: Serious amateurs aiming for high placings within their age categories.
- Finisher-Focused Runners: Individuals whose primary goal is to complete the challenging distance, embracing the personal journey and community aspect.
- Adventure Runners: Those drawn primarily to the exploration of remote terrains and multi-day challenges.
This diversity in goals, abilities, and event types underscores why personalized approaches to training and racing are so crucial.
2.2. Relevant AI Concepts Explained
Artificial intelligence is a broad field. For understanding its role in ultramarathons, a few key concepts are particularly relevant:
2.2.1. What is Artificial Intelligence (AI)?
At its core, AI involves creating computer systems that can perform tasks typically requiring human intelligence. This includes learning from experience, recognizing patterns, making predictions, understanding language, and solving complex problems. It’s not about creating sentient machines like in science fiction, but rather about developing tools that can process information and perform specific tasks intelligently.
2.2.2. Machine Learning (ML): Learning from Data
Machine learning is a subset of AI focused on building systems that can learn from and make decisions based on data, without being explicitly programmed for every single scenario. ML algorithms identify patterns and relationships within large datasets. In the context of running:
- Supervised Learning: The algorithm learns from labeled data (e.g., training data labeled with performance outcomes or injury occurrences) to make predictions or classifications (e.g., predicting race time based on training history, classifying if a runner is at high risk of injury).
- Unsupervised Learning: The algorithm explores unlabeled data to find hidden structures or patterns (e.g., grouping runners with similar physiological profiles or training responses).
- Reinforcement Learning: The algorithm learns by trial and error, receiving rewards or penalties for its actions (e.g., an AI coach learning the optimal training suggestion sequence to maximize long-term fitness gains).
ML is powerful because it can uncover complex, non-linear relationships in physiological data that traditional statistical methods might miss.
2.2.3. Deep Learning (DL): Unlocking Complex Patterns
Deep learning is a specialized type of machine learning that uses artificial neural networks with multiple layers (hence “deep”) to analyze intricate patterns in large datasets. It excels at tasks like image recognition (analyzing running form from video) and natural language processing (understanding text or spoken language, like analyzing race reports or coaching feedback).
2.2.4. Data Analytics & Predictive Modeling
This involves using AI and statistical techniques to analyze historical and current data to identify trends, generate insights, and make predictions about future events. For ultramarathoners, this could mean predicting race finish times based on training data, forecasting fatigue levels, or estimating the likelihood of injury based on training load and biomechanics.
2.2.5. Sensors & the Internet of Things (IoT): The Data Pipeline
AI relies on data. In running, this data primarily comes from sensors embedded in wearable devices (watches, heart rate monitors, running pods) and smartphones. These sensors capture GPS tracks, heart rate, heart rate variability (HRV), running power, cadence, ground contact time, sleep patterns, blood oxygen saturation, and more. Environmental sensors can add data on temperature, humidity, and altitude. The IoT connects these devices, allowing data to be collected, aggregated, and transmitted for analysis by AI algorithms, often in cloud-based platforms.
2.2.6. Computer Vision and NLP in Running
- Computer Vision: AI techniques that enable computers to “see” and interpret images or videos. In running, this is increasingly used for automated gait analysis from simple video recordings.
- Natural Language Processing (NLP): AI techniques focused on understanding and processing human language. Potential applications include analyzing athlete journals, extracting insights from race reports, or powering AI chatbot coaches.
With these foundational concepts in place, we can now explore how AI is specifically being applied to revolutionize ultramarathon training.
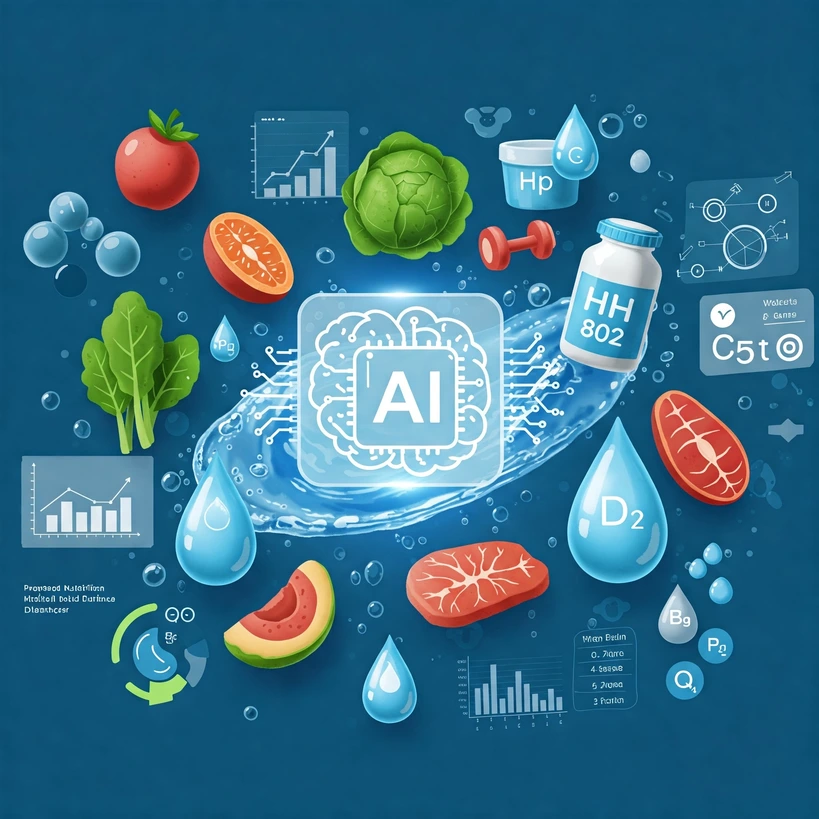
3. The Training Revolution: AI-Powered Preparation
Training for an ultramarathon is a monumental undertaking, requiring months, sometimes years, of dedicated effort. It involves balancing high mileage with adequate recovery, strength work, nutrition, and mental preparation. Traditionally, training plans were often generic, based on established principles but lacking deep individualization. Artificial intelligence is dramatically changing this paradigm, ushering in an era of hyper-personalized, data-driven, and dynamically adaptive training preparation.
3.1. The Data Ecosystem: Fueling the AI Engine
The effectiveness of AI in training hinges on the quality and quantity of data it can access. Modern runners generate a torrent of data, creating a rich ecosystem for AI analysis.
3.1.1. Wearable Technologies: The Personal Data Stream
The primary data source is the array of sensors packed into modern running watches, chest straps, and running pods. These devices capture a wealth of physiological and biomechanical metrics, including:
- GPS Data: Distance, pace, speed, elevation gain/loss.
- Heart Rate (HR): Measures cardiovascular effort during exercise and rest.
- Heart Rate Variability (HRV): The variation in time between consecutive heartbeats, a key indicator of autonomic nervous system balance, recovery status, and physiological stress.
- Running Power: An estimate of the metabolic cost of running, accounting for pace, gradient, and sometimes wind.
- Running Dynamics: Cadence (steps per minute), ground contact time, vertical oscillation (bounce), stride length.
- Sleep Tracking: Duration, sleep stages (light, deep, REM), interruptions, quality scores.
- Other Metrics: Blood oxygen saturation (SpO2), respiration rate, skin temperature (less common).
Platforms associated with these devices (e.g., Garmin Connect, Coros Training Hub, Polar Flow, Suunto App) often perform initial data processing and provide basic insights.
3.1.2. Environmental & Manual Inputs
Beyond automated sensor data, AI systems can incorporate:
- Environmental Data: Weather conditions (temperature, humidity, wind), altitude, and terrain data (from mapped routes) significantly impact effort and performance.
- Manual Inputs: Subjective feedback like Rated Perceived Exertion (RPE) for workouts, nutrition and hydration logs, mood, stress levels, and muscle soreness provide crucial context that sensors alone cannot capture.
3.1.3. Data Platforms & Integration Challenges
Bringing all this disparate data together into a unified platform for AI analysis is a key challenge. Many dedicated AI training platforms (discussed later) aim to integrate data from various sources like Garmin, Strava, Suunto, Apple Health, and TrainingPeaks to create a holistic view of the athlete. Ensuring data accuracy, consistency, and seamless integration across different devices and platforms remains an ongoing area of development.
3.2. Personalized Training Plans: Beyond One-Size-Fits-All
Perhaps the most significant impact of AI in training is its ability to move beyond generic templates and create truly personalized plans. Recognizing that every athlete is unique, AI leverages the collected data to tailor training specifically to the individual.
3.2.1. AI Profiling: Understanding the Individual Runner
AI algorithms analyze an athlete’s historical training data, performance results, physiological metrics (like VO2 Max estimates or threshold paces derived from HRV like DFA alpha 1), and potentially even biomechanical data to build a detailed profile. This profile identifies:
- Strengths and Weaknesses: Is the runner stronger on climbs or flats? Is their aerobic base solid, but speed lacking?
- Training Response: How does the athlete typically respond to different types of workouts (e.g., high intensity vs. volume)? How quickly do they recover?
- Optimal Training Load: What volume and intensity combination yields the best adaptations without leading to excessive fatigue or injury?
3.2.2. Dynamic Adaptation: Training That Lives and Breathes
A key differentiator of AI-driven training is its dynamic nature. Unlike static plans downloaded or written months in advance, AI plans can adapt in near real-time based on the athlete’s ongoing progress and feedback.
- Daily Adjustments: If an athlete has a poor night’s sleep, shows a concerning drop in HRV indicating low recovery, or reports high stress levels, the AI can automatically adjust the day’s planned workout – perhaps suggesting an easier session, a rest day, or swapping workout types. Conversely, if the athlete is responding exceptionally well, the AI might cautiously increase the challenge.
- Adapting to Missed Sessions: If life gets in the way and a workout is missed, the AI can recalibrate the following days or week to keep the overall training progression on track without simply skipping crucial stimulus.
- Environmental Factors: Some platforms can factor in forecast weather conditions or altitude changes, adjusting planned efforts accordingly.
This constant feedback loop ensures the training remains appropriate and effective, optimizing the balance between pushing boundaries and ensuring adequate recovery – a critical tightrope walk in ultramarathon preparation. Platforms like Humango, TrainAsONE, and Athletica AI emphasize this adaptive capability.
3.2.3. Optimizing Periodization and Load Management
Periodization involves structuring training into cycles (macro, meso, micro) with varying focus, intensity, and volume to peak for specific events. AI can assist in optimizing these cycles based on the athlete’s profile, goals, and response to training. It can help ensure a progressive overload that stimulates adaptation while minimizing the risk of overtraining or burnout, which is particularly crucial during the high-volume blocks typical of ultramarathon training. AI can analyze metrics related to training stress balance (TSB), chronic training load (CTL), and acute training load (ATL) to provide data-driven guidance on load management.
3.2.4. Specific Workout Recommendations
Based on the overall plan and the athlete’s daily readiness, AI can recommend specific workouts, detailing duration, intensity (based on heart rate, power, or pace zones – which themselves can be dynamically determined by AI using HRV/DFA alpha 1), and structure (e.g., interval lengths and rest periods). This ensures each session serves a purpose within the larger strategy.
3.3. Performance Prediction & Goal Setting
Beyond planning workouts, AI’s predictive capabilities offer significant advantages for ultramarathoners.
3.3.1. Forecasting Race Outcomes
By analyzing an athlete’s training history, current fitness metrics, and potentially course characteristics, AI algorithms can predict potential race times or performance levels for various distances. Platforms like AI Endurance explicitly offer race pace predictors. While these predictions come with a margin of error dependent on data quality and quantity, they provide valuable, data-driven benchmarks. Studies have even used machine learning (like XG Boost) to predict ultramarathon running speed based on factors like age, sex, origin, race location, type, and surface.
3.3.2. Identifying Performance Limiters
AI analysis can help pinpoint the specific factors holding a runner back. Is it aerobic capacity, muscular endurance on climbs, running economy, or perhaps insufficient recovery? Identifying these limiters allows training to be focused more effectively. AI Endurance, for example, focuses on optimizing “ultra endurance power,” defined as the power at a runner’s aerobic threshold, identified using HRV technology (DFA alpha 1).
3.3.3. Data-Informed Goal Setting
AI-driven performance predictions allow runners to set more realistic and achievable goals for upcoming races. This can be highly motivating and helps structure training appropriately. For ultramarathoners, where preparation is long and race opportunities might be infrequent, having data-based feedback on progress towards a goal pace or time is invaluable.
3.4. Technique and Form Analysis: The AI Biomechanist
Efficient running form is crucial for ultramarathoners to conserve energy over long distances and reduce the risk of overuse injuries. Traditionally, gait analysis required expensive laboratory equipment and expert interpretation. AI, particularly computer vision, is democratizing this process.
3.4.1. Computer Vision for Gait Analysis
Platforms like Couro and Ochy utilize AI to analyze running form from simple video recordings taken with a smartphone or action camera. The AI identifies key body landmarks and tracks their movement, calculating various biomechanical metrics.
3.4.2. Sensor-Based Biomechanical Measurement
Running dynamics pods (worn on the shoe or waist) and smart insoles capture metrics like:
- Cadence
- Ground Contact Time (GCT)
- GCT Balance (Left vs. Right)
- Vertical Oscillation
- Stride Length
- Running Power
AI algorithms can analyze these sensor-derived metrics to identify potential inefficiencies or asymmetries.
3.4.3. AI-Driven Feedback for Efficiency and Prevention
AI gait analysis systems can measure key metrics and compare them to optimal ranges or the runner’s own baseline. More importantly, platforms like Ochy go beyond data provision to offer personalized recommendations and improvement plans based on the AI analysis. This might include specific running drills or strengthening exercises designed to address identified weaknesses, imbalances (asymmetries), or potentially injury-prone movement patterns.
For ultramarathoners, who are highly susceptible to overuse injuries due to high training volume, accessible AI-driven gait analysis offers a powerful tool for proactively identifying and addressing biomechanical issues. Optimizing running form can lead to reduced injury risk, improved running economy (energy conservation), and ultimately, enhanced performance over ultra distances.
The integration of AI into training preparation marks a significant shift, moving from static, generalized plans towards dynamic, hyper-personalized, and continuously optimized strategies informed by a wealth of individual data. This revolution holds immense promise for helping ultramarathoners train smarter, stay healthier, and reach new levels of performance.
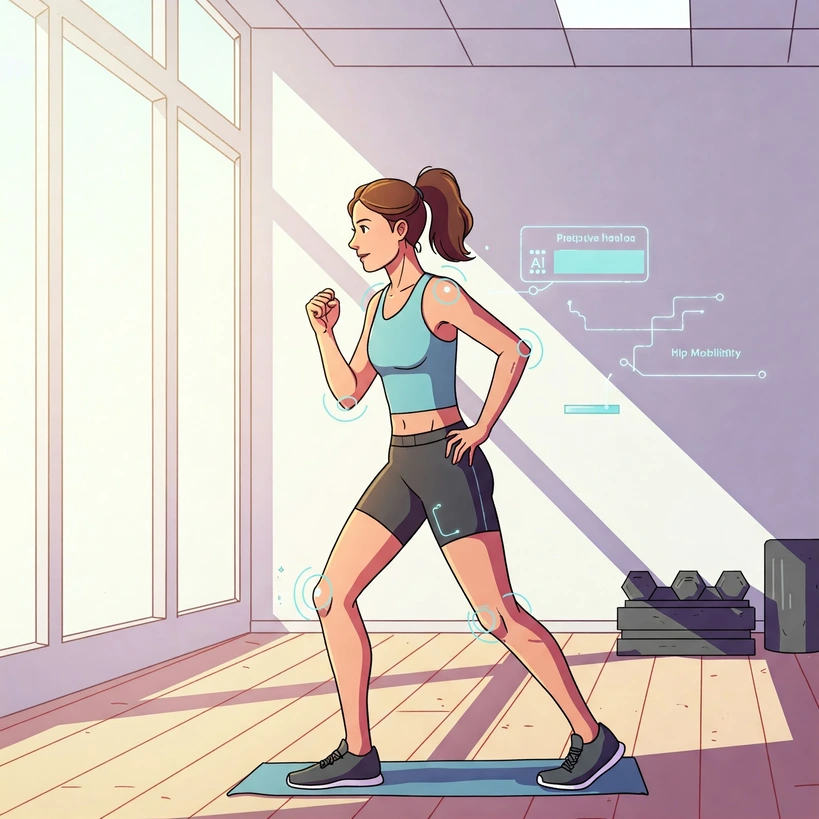
4. Intelligent Fueling: AI for Nutrition & Hydration Optimization
Fueling and hydration are arguably among the most critical—and most challenging—aspects of ultramarathon running. The extreme duration and intensity demand enormous energy expenditure and can lead to significant fluid and electrolyte loss. Mistakes in fueling strategy frequently lead to performance collapse (the dreaded “bonk” or hitting the wall due to glycogen depletion), debilitating gastrointestinal (GI) distress, dehydration, or dangerous electrolyte imbalances like hyponatremia. Artificial intelligence is emerging as a valuable tool to help athletes navigate these complex nutritional demands with greater precision and personalization.
4.1. Deep Dive into Individual Needs
Generic nutrition guidelines often fall short for ultramarathoners due to vast individual differences in metabolism, sweat rates, and specific race conditions. AI offers the potential to provide far more tailored recommendations.
4.1.1. Estimating Energy Expenditure
AI algorithms can analyze data from wearables (activity levels, heart rate, power output) combined with athlete profile information (age, weight, sex, fitness level) to provide more accurate estimates of caloric expenditure during training and racing. Some platforms, like AI Endurance, develop algorithms to estimate daily and exercise-specific energy needs based on the individual’s training routine. Understanding these needs is the first step towards developing an adequate fueling plan.
4.1.2. Sweat Analysis & Electrolyte Loss Prediction
Individual sweat rates and, crucially, the concentration of electrolytes (particularly sodium) lost in sweat vary dramatically between athletes. This variability makes personalized hydration and electrolyte replacement strategies essential.
- AI-Powered Sweat Testing: Companies like Precision Hydration offer sweat testing services to measure sodium loss. While the testing itself may not be AI-driven, AI could potentially be used to analyze results alongside other athlete data (training load, environment) to refine personalized hydration plans. Emerging sensor technologies aim to analyze sweat composition in real-time, and AI would be crucial for interpreting this data stream effectively.
- Predictive Models: Research is underway to develop AI algorithms that can predict an athlete’s whole-body sweat rate based on inputs like environmental conditions (temperature, humidity) and activity details (intensity, duration). Such models could help athletes anticipate fluid needs before outdoor training sessions or races.
AI-driven insights into sweat loss allow athletes to move beyond guesswork and tailor their fluid and electrolyte intake (e.g., sodium concentration in drinks or salt tabs) with much greater precision. This is particularly valuable for ultramarathoners who face prolonged periods of sweating in potentially diverse environmental conditions.
4.1.3. Determining Macro/Micronutrient Needs
AI can analyze an athlete’s training load, goals, body composition, and even dietary preferences to recommend optimal macronutrient intake (carbohydrates, proteins, fats) and timing. AI Endurance, for example, provides recommendations for key macronutrients alongside energy requirements. This helps ensure athletes consume enough carbohydrates to fuel activity and replenish glycogen stores, sufficient protein for muscle repair and adaptation, and adequate healthy fats for overall health.
4.2. In-Training and Race Day Strategies
Developing a workable fueling plan for an ultramarathon, where athletes might be running for 10, 20, 30 hours or more, is incredibly complex. AI offers potential solutions for creating and adapting these strategies.
4.2.1. Dynamic Fueling Plans: Precision Energy Delivery
AI could potentially synthesize data on the athlete’s real-time effort (pace, heart rate, power), the course profile (upcoming climbs), environmental conditions, and pre-race nutritional status to generate dynamic fueling recommendations during an event. The goal would be to maintain stable energy levels, prevent glycogen depletion, and minimize GI distress. This might involve suggesting specific amounts of carbohydrates, fluids, and electrolytes at optimal intervals.
4.2.2. Real-time Recommendations & Alerts (The Potential)
While perhaps not yet widely implemented in commercially available race-day apps, the future could see AI providing real-time prompts via a watch or phone: “Consume 30g carbs now,” “Increase fluid intake on the next climb,” or “Electrolyte levels may be dropping based on predicted sweat loss.” Large language models (LLMs) like ChatGPT-4 have already demonstrated notable proficiency in sports nutrition knowledge, sometimes outperforming experienced athletes and even registered dietitians in assessments, suggesting the potential for AI to serve as a powerful resource for nutritional advice and education. However, expert human judgment remains crucial for clinical reasoning and fully personalized assessments based on specific health conditions.
4.2.3. Continuous Glucose Monitoring (CGM) Integration
CGMs provide real-time data on blood glucose levels. Integrating this data stream with AI could allow for highly precise, individualized carbohydrate intake recommendations during training and racing, helping athletes maintain stable blood sugar and avoid energy crashes. AI could learn an individual’s glucose response to different types and amounts of fuel under various exercise intensities.
4.3. Nutrition Tracking and Impact Analysis
Understanding the relationship between nutrition and performance requires consistent tracking and analysis, areas where AI can provide significant assistance.
4.3.1. AI-Powered Food Logging
Manually logging food intake can be tedious. AI is improving this process through:
- Image Recognition: Apps that allow users to take photos of their meals, with AI identifying the food items and estimating portion sizes.
- Natural Language Processing: Allowing users to dictate their meals or type descriptions naturally for the AI to parse and log.
4.3.2. Correlating Diet with Performance and Recovery
Once dietary data is logged alongside training data (performance metrics, RPE) and recovery data (sleep, HRV), AI algorithms can analyze correlations. Did a specific pre-race meal lead to better performance? Does higher protein intake correlate with faster recovery (as measured by HRV)? These insights can help athletes refine their nutritional strategies based on objective data linking diet to outcomes.
For ultramarathon runners facing enormous caloric demands and the high risk of fueling-related issues, AI-driven nutrition and hydration strategies offer the potential for highly customized, data-informed plans. By helping runners maintain energy levels, prevent bonking, minimize GI problems, and stay adequately hydrated with proper electrolyte balance, AI can significantly contribute to optimizing performance, improving recovery, and enhancing overall safety during these extreme endurance events.
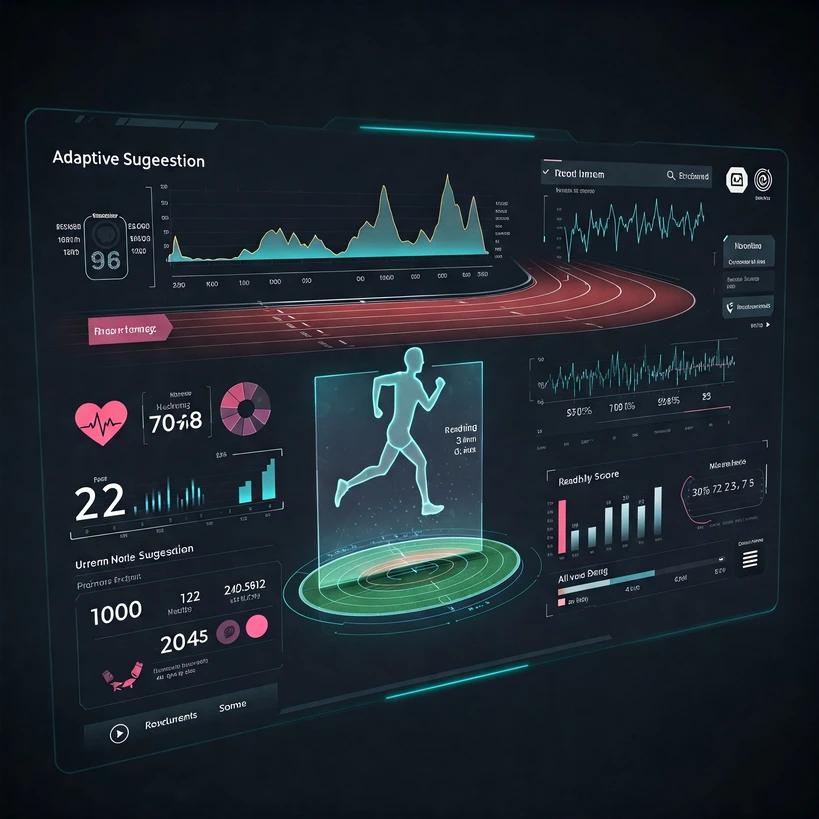
5. Combating Injuries: AI for Prediction and Prevention
Injuries are the bane of many runners, particularly ultramarathoners whose high training volumes and the repetitive nature of their sport place immense stress on the musculoskeletal system. Overuse injuries like stress fractures, tendinopathies (e.g., Achilles, patellar), plantar fasciitis, and runner’s knee are common. Traditionally, injury management has been reactive – dealing with injuries after they occur. Artificial intelligence offers the exciting potential to shift towards a proactive approach, identifying athletes at risk before an injury fully manifests and allowing for preventative interventions.
5.1. Identifying Risk Factors: Beyond Gut Feeling
AI excels at analyzing complex datasets to identify subtle patterns that might indicate an elevated injury risk. These patterns can emerge from various data streams:
5.1.1. Analyzing Training Load Patterns
Overtraining or inappropriate load management is a major contributor to running injuries. AI algorithms can analyze metrics like:
- Acute:Chronic Workload Ratio (ACWR): Comparing recent training load (acute) to longer-term load (chronic). Sudden spikes in this ratio are often associated with increased injury risk.
- Training Monotony: Measuring the lack of variation in daily training loads, which can also increase risk.
- Training Strain: Quantifying the overall stress imposed by training sessions.
AI can identify dangerous trends in these metrics that might otherwise go unnoticed.
5.1.2. Biomechanical Data Insights
As discussed in the training section, AI-driven gait analysis (using computer vision or sensors) can detect biomechanical inefficiencies, asymmetries, or abnormal movement patterns. These deviations from optimal form can place excessive stress on specific tissues, predisposing the runner to injury. AI can flag these potentially harmful patterns.
5.1.3. Physiological Stress Indicators (HRV, Sleep)
Data from wearables can provide insights into the body’s overall stress and recovery state. Consistently low Heart Rate Variability (HRV) or poor sleep quality can indicate that the body is under excessive physiological stress and may be less resilient to training loads, thus increasing injury susceptibility. AI models can track these trends over time.
5.2. Injury Prediction Models: A Proactive Approach
Leveraging the ability to identify risk factors, researchers and developers are building AI models specifically designed to predict injury likelihood.
5.2.1. Machine Learning for Risk Scoring
Machine learning techniques, including random forests, support vector machines, and neural networks, are proving effective in predicting injury risk. These algorithms can learn complex, non-linear relationships between various risk factors (training load, biomechanics, previous injury history, physiological data, even psychological factors) and the probability of sustaining an injury. The output might be a personalized risk score or classification (e.g., low, medium, high risk).
5.2.2. Forecasting Specific Injury Types
Future models might even become sophisticated enough to predict the risk of specific types of injuries based on an individual’s unique profile and data patterns. For example, certain gait abnormalities might be strongly correlated with runner’s knee, while specific training load patterns might predict stress fractures.
5.3. Early Warning Systems & Preventative Interventions
The true value of predictive modeling lies in its ability to enable timely interventions.
5.3.1. Alerting Athletes and Coaches
When an AI system detects a significant increase in an athlete’s injury risk score or flags a concerning pattern (e.g., a sudden drop in HRV combined with a spike in training load), it can generate alerts for the runner and/or their coach. This provides an objective, data-driven heads-up before subjective symptoms like pain might even appear.
5.3.2. AI-Suggested Modifications
Based on the identified risk factors, AI platforms can suggest specific preventative actions:
- Training Adjustments: Recommending reduced training volume or intensity, incorporating more rest days, or modifying specific workout types.
- Biomechanical Corrections: Suggesting specific drills, form cues, or strengthening exercises to address gait inefficiencies identified through AI analysis.
- Recovery Enhancement: Advising on strategies to improve sleep quality or manage stress based on physiological data.
This allows for a proactive, personalized approach to injury prevention, tailored to the individual’s specific needs and risk profile.
5.4. Supporting Rehabilitation
While the primary focus is prevention, AI also has potential applications in injury rehabilitation:
5.4.1. Monitoring Recovery Progress
Wearable data (e.g., HRV, sleep quality, movement patterns) can be analyzed by AI to objectively track an athlete’s recovery progress after an injury, helping guide the return-to-running process.
5.4.2. Guiding Rehab Exercises (Future Potential)
Computer vision could potentially be used to monitor patients performing rehabilitation exercises at home, providing feedback on form and adherence to the prescribed program, similar to its application in gait analysis. Physical therapists could use AI analysis of movement during rehab to tailor programs more effectively.
For ultramarathon runners, who place enormous and repetitive stress on their bodies and for whom lengthy injury layoffs can be devastating to long-term goals, the predictive and preventative capabilities of AI offer a significant advantage. By leveraging AI models, runners and coaches can gain valuable insights into potential risks, enabling proactive adjustments to training load, intensity, biomechanics, and recovery protocols. This data-driven approach can help minimize the likelihood of setbacks, promote long-term sustainability in the sport, allow for more consistent training, and ultimately help runners achieve their performance goals more reliably. AI’s ability to analyze individual data and predict risks before they manifest could be a true game-changer for managing the high injury susceptibility inherent in ultramarathon training.
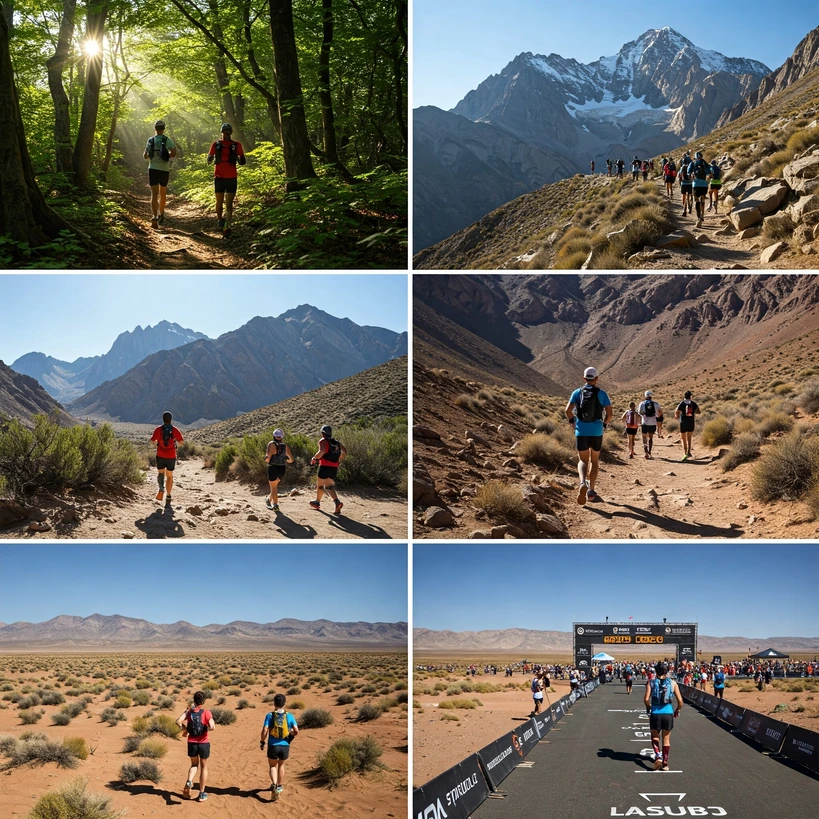
6. Race Day Intelligence: AI Strategy and Real-time Support
Race day for an ultramarathon is the culmination of months of training, but executing effectively over such extreme distances presents its own unique set of challenges. Pacing, fueling, hydration, and adapting to changing conditions are critical for success. While AI is making significant strides in training preparation, its application in providing real-time guidance during an ultramarathon itself is currently less developed but holds immense future potential.
6.1. Crafting the Optimal Race Strategy
Before the race even begins, AI can play a role in formulating a data-driven strategy.
6.1.1. Course Profile and Terrain Analysis
AI can analyze detailed course maps, including elevation profiles and known terrain types (technical singletrack, runnable fire roads, steep climbs/descents). Combined with the runner’s historical performance data on similar terrain (gleaned from training logs), AI could help predict how challenging specific sections will be for that individual.
6.1.2. Weather Condition Integration
Factoring in the predicted weather forecast (temperature, humidity, wind, precipitation) for race day allows AI to estimate the likely impact on performance and physiological needs (e.g., increased fluid requirements in heat).
6.1.3. Personalized Pacing Plans Pre-Race
Based on the course analysis, weather forecast, the runner’s fitness level (derived from AI analysis of training data and performance predictions), and their target goal, AI can help generate a detailed, personalized pacing strategy before the race. This might involve target pace ranges, heart rate zones, or power targets for different sections of the course. Common ultramarathon strategies like aiming for a “negative split” (running the second half faster than the first to conserve energy early) could be modeled and personalized using AI. However, strict adherence to pace alone can be problematic in ultras due to terrain variability and physiological drift over time. Many experienced ultrarunners rely more on Rate of Perceived Exertion (RPE), aiming to stay below aerobic/lactate thresholds early on to preserve glycogen. AI could potentially help translate a target RPE strategy into suggested pace/HR/power ranges for different course segments. Research on track-based ultras suggests consistency is key – the most successful runners slow down the least over the event’s duration.
6.2. Real-time Applications: The Future Horizon
The truly revolutionary potential lies in AI providing dynamic, adaptive guidance during the race itself. While current implementations are limited, the capacity of AI to process multiple real-time data streams suggests significant future possibilities.
6.2.1. Dynamic Pace Adjustments: The AI Coach Vision
Imagine an AI coach integrated into a running watch or app providing real-time pacing suggestions based on:
- Current Physiological Data: Heart rate, running power, potentially HRV trends or other fatigue indicators.
- Progress vs. Plan: Comparing current pace/effort to the pre-race strategy.
- Upcoming Terrain: Adjusting recommended effort for imminent climbs or descents.
- Environmental Conditions: Factoring in current temperature or wind shifts.
- Goal Time: Calculating necessary adjustments to stay on track for the target finish.
This AI could advise when to push harder, when to conserve energy, or suggest specific adjustments to maintain an optimal effort level throughout the race’s changing demands.
6.2.2. Predicting Fatigue and “Hitting the Wall”
By analyzing trends in heart rate (e.g., cardiac drift – HR increasing at the same pace), pace degradation, running power changes, or potentially biomechanical shifts (detected via pods), AI might be able to predict impending severe fatigue or glycogen depletion (“bonking”) before the runner fully experiences it. Early warnings could prompt preventative actions like adjusting pace or increasing fuel intake.
6.2.3. Intelligent Nutrition/Hydration Prompts
Based on elapsed time, intensity, predicted sweat rate (factoring in real-time conditions), and potentially CGM data, an AI system could provide personalized reminders for fluid, electrolyte, and calorie intake during the race, helping athletes stay on top of their fueling plan even when mentally fatigued.
6.2.4. Enhanced Navigation and Course Management
For trail ultramarathons, AI could potentially enhance navigation features on watches, perhaps offering dynamic re-routing suggestions if a section of the course becomes unexpectedly hazardous or if the runner needs to divert to a closer aid station based on their condition (though race rules often restrict leaving the marked course).
Current Reality and Challenges:
It’s crucial to reiterate that sophisticated, real-time AI race guidance for ultramarathons is largely futuristic. Challenges include:
- Computational Power: Performing complex AI analysis directly on a watch with limited battery life.
- Data Accuracy: Ensuring real-time sensor data (especially physiological metrics under duress) is reliable.
- Connectivity: Cloud-based AI requires reliable data connectivity, often unavailable in remote ultra locations.
- Individual Variability: Accurately modeling the complex interplay of factors influencing performance over 10+ hours is incredibly difficult.
- The Human Element: Over-reliance on technology could potentially disconnect runners from their own bodily intuition and RPE, which remain vital skills in ultramarathing.
Despite these hurdles, the potential for AI to provide data-driven, adaptive support during the grueling hours of an ultramarathon is immense. As technology evolves, we can expect to see increasingly sophisticated AI features aimed at helping runners navigate the complexities of race day execution more effectively.
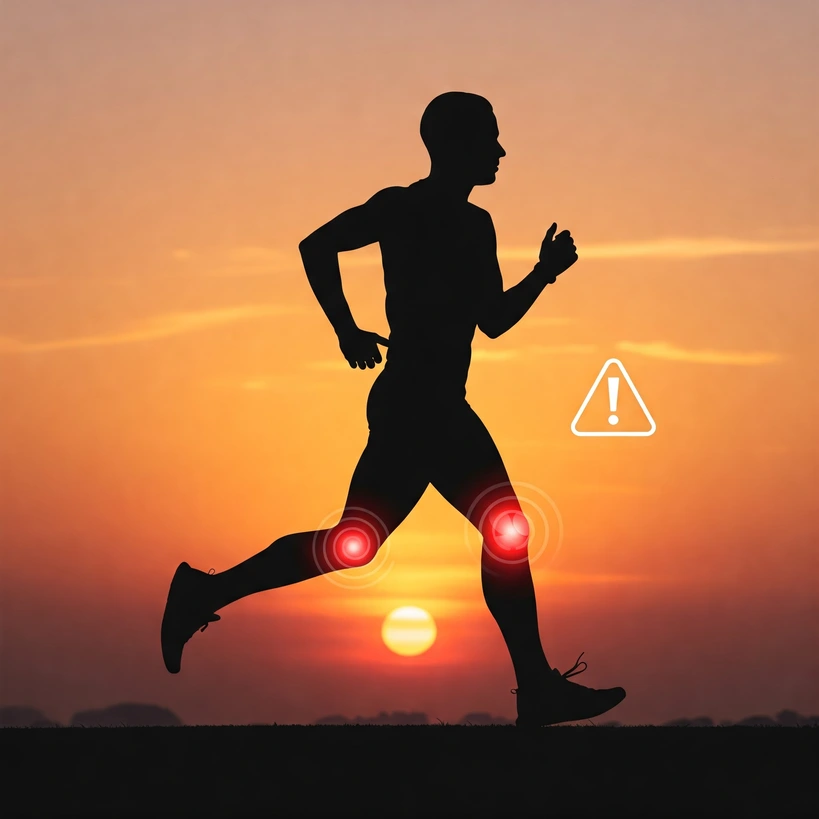
7. The Art of Recovery: AI-Driven Optimization
Recovery is not just the absence of training; it’s an active process where the body repairs damage, replenishes energy stores, and adapts to the imposed stress, ultimately leading to improved fitness. For ultramarathoners subjecting their bodies to extreme and prolonged stress, optimizing recovery is absolutely critical for sustaining high training loads, preventing injuries, and maximizing performance gains. Artificial intelligence is providing powerful new tools to objectively monitor recovery status and guide personalized recovery strategies.
7.1. Monitoring Recovery Status Objectively
Traditionally, athletes relied heavily on subjective feelings (“How tired do I feel?”) to gauge recovery. While important, subjective perception can be unreliable. AI leverages objective data from wearables to provide a clearer picture.
7.1.1. Analyzing Sleep Quality and Stages
Sleep is arguably the most critical recovery tool. AI algorithms analyze data from watch sensors (accelerometers, heart rate monitors) to estimate:
- Sleep Duration: Total time asleep.
- Sleep Stages: Time spent in Light, Deep, and REM sleep, each playing different roles in physical and mental restoration.
- Sleep Quality: Metrics incorporating duration, stages, restlessness, and interruptions.
AI platforms can track sleep trends over time, correlating them with training load and performance, and provide insights into how sleep habits impact recovery.
7.1.2. Tracking Heart Rate Variability (HRV) Trends
HRV has emerged as a key objective marker of physiological stress and recovery. It reflects the balance of the autonomic nervous system (ANS), which controls involuntary bodily functions.
- High HRV (Relative to Baseline): Generally indicates good recovery, lower stress, and readiness for training (parasympathetic dominance).
- Low HRV (Relative to Baseline): Often suggests accumulated fatigue, high stress (physical or mental), potential illness, or poor recovery (sympathetic dominance).
AI algorithms are crucial for interpreting HRV data effectively. They establish an individual’s baseline HRV and then track daily fluctuations and longer-term trends, providing a more nuanced understanding than a single daily reading. AI can filter out noise and identify meaningful deviations from the norm.
7.1.3. Monitoring Resting Heart Rate (RHR)
While less sensitive than HRV, trends in RHR can also indicate recovery status. An elevated RHR compared to baseline can sometimes signal fatigue, dehydration, or impending illness. AI can track these trends alongside other metrics.
7.2. Personalized Recovery Protocols
Based on the objective monitoring of recovery status, AI can help tailor recovery strategies to the individual’s needs on any given day.
7.2.1. Recommending Optimal Rest & Active Recovery
If AI analysis of HRV, sleep, and training load indicates poor recovery, the system might recommend:
- Passive Rest: Taking a complete rest day or significantly reducing planned activity.
- Active Recovery: Suggesting low-intensity activities like walking, stretching, or yoga to promote blood flow without adding significant stress.
- Modifying Training: Automatically adjusting the next day’s training plan to be less intense or shorter.
7.2.2. AI-Driven Sleep Improvement Advice
Based on sleep tracking analysis, AI might offer personalized tips to improve sleep hygiene, such as suggesting optimal bedtimes, recommending pre-sleep routines, or identifying factors (like late-day caffeine or intense evening workouts) that might be negatively impacting sleep quality.
7.2.3. Stress Management Guidance
Since mental stress impacts physiological recovery (reflected in HRV), some AI platforms might integrate stress tracking (based on HRV or user input) and suggest mindfulness exercises, breathing techniques, or other stress-reduction strategies when high stress levels appear to be hindering recovery.
7.3. Training Readiness Scores: Go or Hold Back?
Many AI platforms and wearables synthesize various recovery metrics (HRV, sleep, recent training load, RPE) into a single, easy-to-understand “Readiness Score” or “Recovery Score.”
- High Readiness: Indicates the body is well-recovered and prepared to handle a challenging training session.
- Low Readiness: Suggests the body needs more recovery, and pushing hard could be counterproductive or increase injury risk.
These AI-driven scores provide athletes with actionable, daily guidance on whether to proceed with planned intense training, opt for an easier session, or prioritize rest. Platforms like AI Endurance utilize HRV data to build personalized recovery models, learning how training stress impacts an individual’s recovery and how long they typically take to bounce back from different workout types.
For ultramarathoners, whose training necessitates imposing significant physiological stress, mastering recovery is non-negotiable. The sheer volume and intensity required demand meticulous attention to rest and regeneration. AI-powered recovery monitoring and guidance provide an invaluable toolset. By offering objective insights into their physiological state and providing personalized recommendations, AI helps ultramarathoners make smarter decisions about their training intensity and volume. This data-driven approach optimizes the adaptation process, minimizes the risks of overtraining and injury associated with inadequate recovery, and ultimately supports both peak performance and long-term health in this demanding sport.
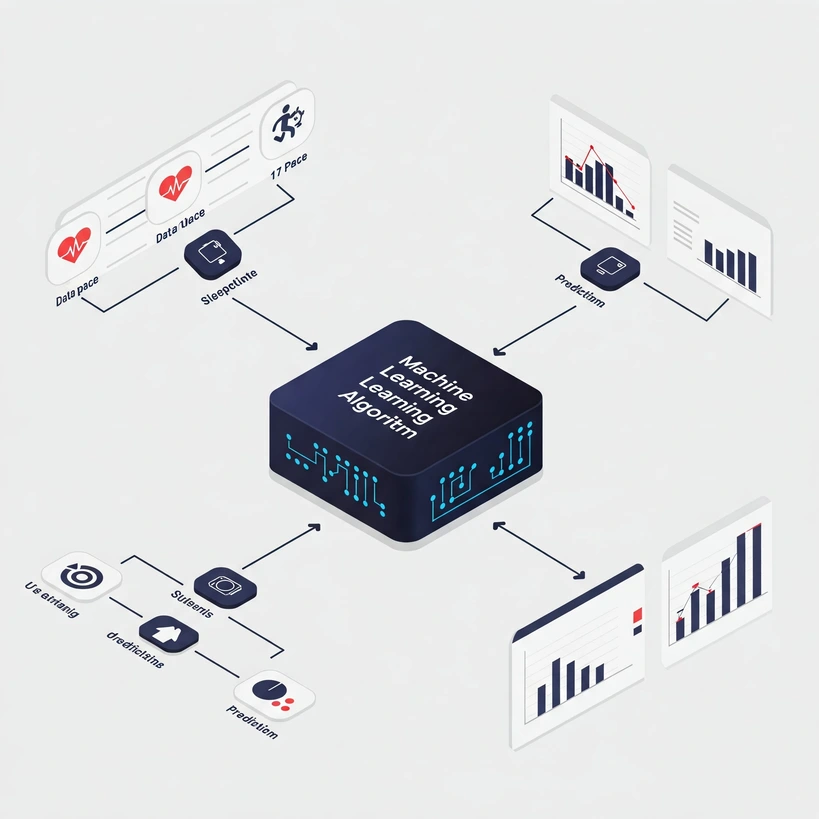
8. Beyond the Athlete: AI for Community, Organizers & Gear
While the most prominent applications of AI in ultramarathon running currently focus on optimizing the individual athlete’s performance and health, the technology’s potential extends to the broader ecosystem surrounding the sport. AI could enhance the experience for spectators, aid race organizers in logistics and safety, influence gear design, and foster stronger community connections.
8.1. Enhanced Spectator Experience
Ultramarathons, especially long trail races through remote areas, can be challenging for spectators to follow. AI could help bridge this gap:
8.1.1. AI-Powered Predictions and Highlights
- Predictive Tracking: AI could analyze runner tracking data, historical splits, and course profiles to predict estimated arrival times at various aid stations or key viewpoints, helping spectators plan where and when to see their runners.
- Automated Highlights: AI algorithms could analyze race data (lead changes, significant pace shifts, finishers) and potentially integrated video feeds to automatically generate race summaries or highlight reels for online followers or event recaps.
- Virtual Spectating: AI could power enhanced virtual race visualizations, offering richer data overlays and predictive insights for those following online.
8.1.2. Personalized Spectator Information
Spectator apps could use AI to provide personalized updates based on the specific runners they are tracking, filtering relevant information and predictions from the noise of the larger event.
8.2. Event Management & Safety
Organizing an ultramarathon, particularly a large trail race, is a complex logistical undertaking with safety as a paramount concern. AI offers potential solutions:
8.2.1. Optimizing Logistics (Aid Stations, Crew)
- Aid Station Stocking: By analyzing historical data, predicted participation numbers, course difficulty, and weather forecasts, AI could help organizers predict resource needs (food, water, medical supplies) at different aid stations more accurately, reducing waste and preventing shortages.
- Volunteer Deployment: AI could assist in optimizing the placement and scheduling of volunteers based on predicted runner flow and peak times at various points on the course.
- Crew Access Planning: For races allowing support crews, AI could potentially analyze traffic patterns and optimal driving routes between crew-accessible aid stations.
8.2.2. AI for Runner Tracking and Safety Alerts
- Enhanced Tracking Analysis: AI can analyze real-time GPS tracking data from all participants more effectively than manual monitoring.
- Anomaly Detection: Algorithms could automatically flag runners who have stopped moving unexpectedly for a prolonged period, gone significantly off-course, or are moving much slower than predicted, potentially indicating distress or injury. This could trigger faster responses from race medical or sweep teams.
- Predictive Safety: By integrating weather data and runner location/speed, AI might identify runners potentially heading into hazardous conditions (e.g., severe storm, nightfall in a technical section unprepared) and alert race management.
8.3. Smart Gear: Beyond Wearable Sensors
AI’s influence is extending into the design and recommendation of running gear itself.
8.3.1. AI in Shoe Design and Material Science
- Simulation & Optimization: AI can be used in the design phase to simulate how different shoe structures, foam compositions, and tread patterns will perform under various conditions and for different runner types (based on weight, foot strike, speed). This can accelerate the development of more efficient, durable, and comfortable footwear.
- Personalized Manufacturing (Future): In the future, AI combined with advanced manufacturing techniques (like 3D printing) could potentially lead to truly personalized running shoes designed based on an individual’s specific biomechanics, gait analysis data, and preferences.
8.3.2. Personalized Equipment Recommendations
AI platforms could analyze a runner’s profile (running style, typical terrain, climate, injury history, past gear preferences) to recommend the most suitable shoes, hydration packs, apparel, or other gear from existing market options.
8.4. Virtual Coaching & Community Platforms
AI is enhancing online running communities and coaching platforms:
- Intelligent Matchmaking: AI could connect runners with similar goals, abilities, or geographical locations within online communities, fostering better peer support and training partnerships.
- Group Data Analysis: For running clubs or groups using integrated platforms, AI could analyze collective training data to identify group trends, organize group runs based on compatible paces, or even facilitate friendly competitions.
- Chatbot Assistants: As seen with WHOOP Coach, AI-powered chatbots can answer common training questions, provide motivation, and offer basic guidance within community forums or apps.
While many of these “beyond the athlete” applications are still in earlier stages of development compared to personalized training AI, they represent a significant area for future growth. As AI technology becomes more accessible and integrated, its impact on the entire ultramarathon experience – for runners, organizers, spectators, and the industry – is likely to become increasingly profound.
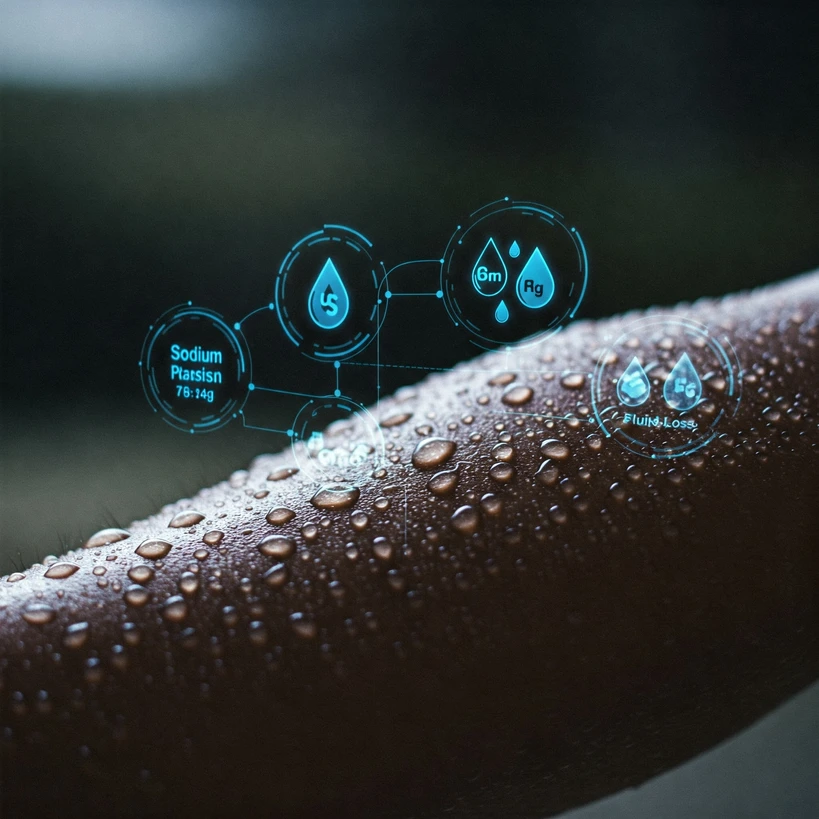
9. Ethical Dimensions, Challenges & Future Perspectives
The integration of artificial intelligence into ultramarathon running, while offering tremendous potential benefits, is not without its challenges and significant ethical considerations. As AI becomes more deeply embedded in how athletes train, compete, and recover, it’s crucial to proactively address these issues to ensure the technology is used responsibly, fairly, and in a way that preserves the integrity and spirit of the sport.
9.1. Data Privacy and Security Concerns
AI systems thrive on data, often highly personal and sensitive data collected from athletes. This raises critical questions:
- Data Ownership: Who owns the vast amounts of physiological, performance, and health data generated by runners – the athlete, the device manufacturer, the platform provider, or the coach?
- Data Security: How securely is this data stored and protected from breaches or unauthorized access?
- Data Usage & Consent: How is the data being used? Are athletes giving informed consent for their data to be used for algorithm training, research, or potentially commercial purposes? Is anonymization sufficient?
Robust security measures, transparent data usage policies, and clear regulations are essential to protect athletes’ privacy and ensure ethical data handling. The potential for misuse, such as creating unfair competitive advantages or discriminating against athletes based on their physiological profiles, must be actively mitigated.
9.2. Algorithmic Bias and Fairness Issues
AI algorithms are trained on data, and if that data reflects existing societal biases or is not representative of the diverse population of runners, the resulting AI systems can perpetuate or even amplify those biases.
- Training Data Bias: Are algorithms trained primarily on data from elite male athletes, potentially making them less accurate or effective for female runners, older runners, or runners from different ethnic backgrounds?
- Unfair Advantages: Could biased algorithms lead to unfair athlete selection, resource allocation, or training recommendations?
- Equal Access: Does the cost or technical knowledge required to use advanced AI tools create an uneven playing field, favoring wealthier or more tech-savvy athletes?
Developing fair, equitable, and rigorously tested algorithms that account for diverse populations is crucial to ensure AI benefits all participants.
9.3. The “Technological Doping” Debate & The Spirit of Sport
As AI provides increasingly sophisticated guidance on training, pacing, and recovery, questions arise about its impact on the fundamental nature of the sport:
- Diminishing the Human Element: Does over-reliance on AI reduce the importance of athlete intuition, self-awareness, experience, and the coach-athlete relationship? Does it turn running into merely following an algorithm?
- Fairness of AI Assistance: Is using advanced AI guidance fundamentally different from having a human coach? At what point does technological assistance cross the line into becoming an unfair advantage, akin to “technological doping”?
- Preserving the Challenge: Ultramarathon running is often celebrated for its raw challenge and the journey of self-discovery. Does optimizing every variable via AI diminish this intrinsic value and the satisfaction derived from overcoming obstacles through personal effort and judgment?
Maintaining a balance between leveraging technology’s benefits and preserving the core human elements of skill, intuition, effort, and the inherent unpredictability of sport is a critical ongoing discussion.
9.4. Accessibility and Cost Barriers
While some AI features are becoming integrated into consumer wearables, the most advanced AI coaching platforms and analytical tools often come with subscription fees. This raises concerns about accessibility:
- Financial Barriers: Can all athletes afford access to the best AI tools, or will this create a divide between those who can pay for optimized guidance and those who cannot?
- Technical Literacy: Does effectively utilizing complex AI platforms require a level of technical understanding that not all runners possess?
Efforts to make AI tools more accessible and user-friendly are needed to prevent the creation of a new technological divide within the sport. The democratization of personalized guidance, moving away from the high cost of traditional one-on-one coaching, is a potential benefit of AI, but only if access is reasonably equitable.
9.5. Limitations of Current Technology
It’s important to acknowledge the current limitations of AI in this field:
- Data Accuracy & Reliability: The quality of AI insights depends heavily on the accuracy of the input data from sensors, which can sometimes be noisy or inaccurate, especially during intense or prolonged activity.
- The “Black Box” Problem: The decision-making processes of some complex AI algorithms (especially deep learning models) can be opaque, making it difficult to understand why a particular recommendation was made. This lack of transparency can hinder trust and make troubleshooting difficult.
- Contextual Understanding: AI may struggle to fully grasp the nuances of an athlete’s life context – external stressors, travel fatigue, minor aches not captured by sensors – that a human coach might easily factor in.
- Over-Simplification: Reducing complex physiological and psychological states to single scores (like “readiness”) can sometimes mask important underlying details.
Users need to be aware of these limitations and use AI insights as a valuable tool alongside, not as a complete replacement for, their own judgment and experience.
9.6. Future Trends: What’s Next for AI in Endurance?
The field is evolving rapidly. We can anticipate several future trends:
- Greater Integration: More seamless integration between wearables, training platforms, nutrition apps, and potentially even environmental data sources.
- Increased Sophistication: Algorithms becoming even better at personalization, prediction, and real-time adaptation.
- Multi-Modal Data Analysis: AI systems incorporating a wider range of data inputs, perhaps including computer vision analysis of form, voice analysis for stress detection, or more advanced biochemical sensors.
- AI for Mental Resilience: Development of AI tools aimed at monitoring psychological stress and providing guidance or exercises to enhance mental fortitude, a crucial aspect of ultramarathon running.
- Enhanced Explainability (XAI): Efforts to make AI decision-making processes more transparent and understandable to users.
- Heightened Ethical Scrutiny: As AI becomes more powerful and pervasive, ethical considerations and regulatory oversight will likely increase.
Addressing the ethical challenges and limitations while continuing to innovate responsibly will be key to unlocking the full positive potential of AI in ultramarathon running and other endurance sports.
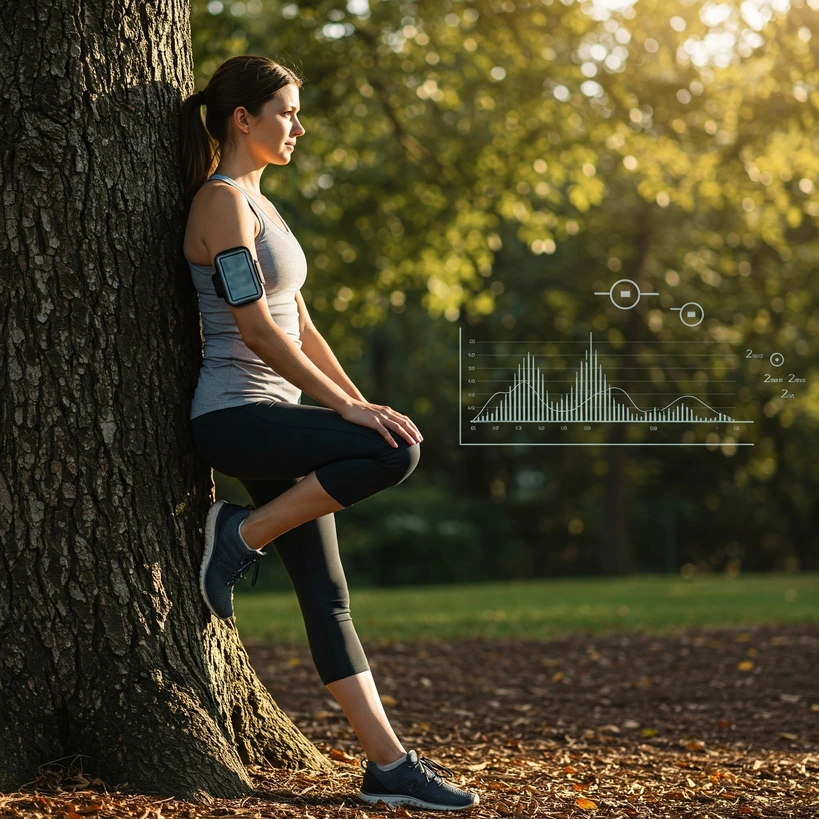
10. Case Studies & Practical Applications
While the theory and potential of AI in ultramarathon running are compelling, seeing how these concepts translate into practice provides valuable context. Although dedicated, peer-reviewed case studies focusing specifically on AI’s impact within the ultramarathon discipline are still emerging, numerous examples, anecdotes, and broader findings illustrate its growing adoption and success in the endurance community.
10.1. Hypothetical Scenarios: AI in Action
To illustrate the potential, consider these hypothetical scenarios:
- Scenario 1: The Injury-Prone Runner: “Sarah” has a history of Achilles tendinopathy flaring up when her mileage increases rapidly. Her AI training platform, analyzing her historical data, current HRV, sleep quality, and recent training load (including data from her running power meter), flags a high injury risk score as she enters a peak volume block. The AI suggests replacing one planned interval session with lower-impact cross-training and slightly reducing the duration of her next long run. It also prompts her to perform specific eccentric calf exercises recommended based on biomechanical data indicating slight asymmetry. Sarah follows the advice, navigates the peak block without injury, and successfully completes her target 100km race.
- Scenario 2: The First-Time 50-Miler: “Mark” is training for his first 50-mile ultramarathon. He feels overwhelmed by the training volume and unsure how to pace himself or fuel properly. He uses an AI coaching app (like Runna or Humango) that integrates with his Garmin watch. The app provides a personalized, adaptive training plan. It adjusts workouts based on his RPE feedback and recovery scores derived from sleep and HRV data. Leading up to the race, the AI provides a detailed pacing strategy based on the course profile and his predicted fitness. During the race (in a future scenario), the AI watch app provides real-time prompts for nutrition intake based on elapsed time and effort level, helping him avoid bonking and finish strong.
- Scenario 3: The Plateaued Veteran: “Maria,” an experienced ultrarunner, feels her performance has plateaued. She uses an AI platform (like AI Endurance or Athletica AI) focused on deeper physiological insights. The AI analyzes her HRV data using DFA alpha 1 to precisely determine her aerobic and anaerobic thresholds. It identifies that while her overall volume is high, she’s spending too little time training near her aerobic threshold, a key factor for ultra-endurance. The platform adjusts her plan to include more specific workouts targeting this intensity zone. It also uses its race predictor to show her potential time improvements, boosting her motivation. Over several months, Maria sees tangible improvements in her threshold power and achieves a new personal best in her next 100-mile race.
10.2. Real-World Examples and Emerging Successes
While detailed, published ultramarathon case studies are sparse, evidence from platform testimonials, reviews, and related research points to practical application:
- Platform User Testimonials: Platforms like TrainAsONE feature user stories, including individuals who successfully prepared for and completed ultra trail marathons using the AI-driven plans. One user described progressing from Lyme disease recovery to finishing their first ultra, highlighting the platform’s ability to provide personalized and adaptive training suited to individual circumstances and goals.
- AI Endurance for 100 Miles: Runner Cat Bradley reportedly used AI Endurance to structure a training plan progressing from 5K fitness to a 100-mile ultramarathon. A review in Trail Runner Magazine noted the AI-generated plans seemed “logical,” suggesting the platform’s capability in handling extreme endurance goals.
- AI vs. Human Coach Effectiveness: A 2022 study published in PLOS One found that AI coaches were as effective as human coaches in helping individuals achieve fitness goals, lending credence to the potential of AI to provide comparable levels of guidance for endurance training.
- Personal Projects & Exploration: There’s growing interest within the running community in experimenting with general AI tools like ChatGPT for creating and adapting training plans. One documented project detailed an individual using ChatGPT as an AI coach to prepare for the 50km “Race to the Stones” ultramarathon, showcasing the perceived potential and increasing accessibility of leveraging AI for personalized training.
- Gait Analysis Adoption: AI-powered gait analysis platforms like Ochy, which secured pre-seed funding and announced integration with the Adidas adiClub program, indicate commercial traction and validation for using AI to analyze running form accessible via smartphone video.
These examples, while varying in rigor, collectively demonstrate that AI is moving beyond theoretical potential and becoming a practical tool used by endurance athletes, including ultramarathoners, to guide their training, manage injury risk, and pursue ambitious goals. As AI technology continues to mature and becomes further integrated into training tools, we can expect more documented case studies and success stories to emerge from the ultramarathon community.
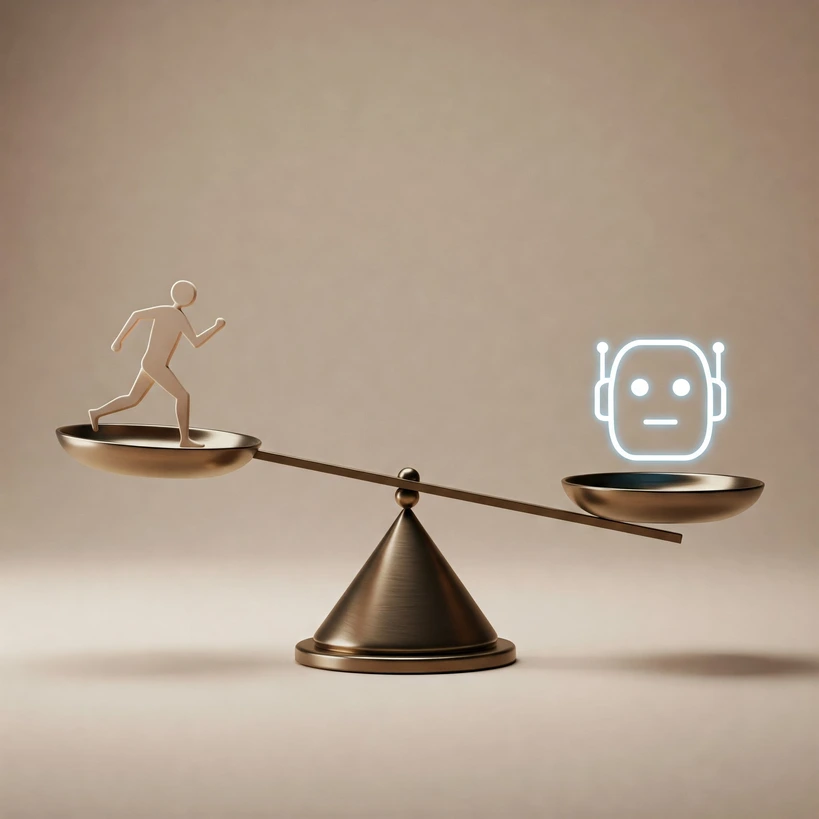
11. Conclusion: The Symbiotic Journey of Human and Machine Endurance
The intersection of artificial intelligence and ultramarathon running represents a compelling frontier in sports science and human performance. As we’ve explored throughout this extensive analysis, AI is no longer a futuristic concept but an increasingly tangible force reshaping how athletes prepare for, execute, and recover from the immense challenges of ultra-distance events.
11.1. Recap of Key Findings
The transformative potential of AI touches nearly every aspect of the ultramarathoner’s journey:
- Training: AI offers hyper-personalized, dynamic training plans that adapt to individual physiology, recovery status, and real-time feedback, moving far beyond static templates. It aids in optimizing training load, periodization, and specific workout prescriptions.
- Performance Prediction: AI analyzes data to provide realistic performance forecasts and helps identify individual limiters, enabling smarter goal setting and more focused training.
- Biomechanics: Accessible AI-driven gait analysis provides insights into running form, helping athletes improve efficiency and proactively address biomechanical flaws that could lead to injury.
- Nutrition & Hydration: AI assists in creating personalized fueling and hydration strategies based on individual needs, estimated energy expenditure, and potentially real-time data like sweat analysis, crucial for managing the complex demands of ultra distances.
- Injury Prevention & Recovery: AI excels at analyzing data (training load, HRV, sleep, biomechanics) to predict injury risk, enabling proactive interventions. It also provides objective monitoring and guidance for optimizing the critical recovery process.
- Wearable Integration: AI is increasingly embedded within smartwatches and fitness trackers, translating raw sensor data into actionable insights readily available to the athlete.
- Coaching Platforms: A growing market of AI-powered coaching platforms offers accessible, data-driven training guidance, democratizing personalized coaching.
11.2. The Balance Between Human Intuition and AI Augmentation
Despite its powerful capabilities, AI is best viewed as a tool to augment, not replace, human intelligence and intuition in ultramarathon running. The experience, self-awareness, mental resilience, and adaptability of the runner remain paramount. Similarly, the nuanced understanding, empathy, and holistic perspective of a human coach are invaluable. The most effective approach likely involves a symbiotic relationship: using AI’s data processing power and analytical insights to inform decisions, while still listening to one’s body, leveraging experience, and potentially collaborating with a human coach who can interpret the data within a broader context.
11.3. Future Outlook: Potential and Responsibilities
The future of AI in ultramarathons promises even greater sophistication and integration. We can expect more refined algorithms, seamless data integration across platforms, and perhaps novel applications targeting mental resilience or real-time race adaptation. However, this progress must be accompanied by a continued focus on ethical considerations – ensuring fairness, transparency, data privacy, and accessibility. The ultramarathon community has a responsibility to guide the adoption of AI in a way that enhances the sport without compromising its core values or the well-being of its participants.
Ultimately, artificial intelligence holds the transformative potential to help ultramarathon runners train smarter, stay healthier, perform better, and push the known limits of human endurance. By embracing data-driven insights and adaptive tools, while remaining mindful of the irreplaceable human element, athletes can embark on a new era of exploration in this incredibly challenging and rewarding sport. The journey of endurance is evolving, and AI is proving to be a powerful companion on the long road ahead.
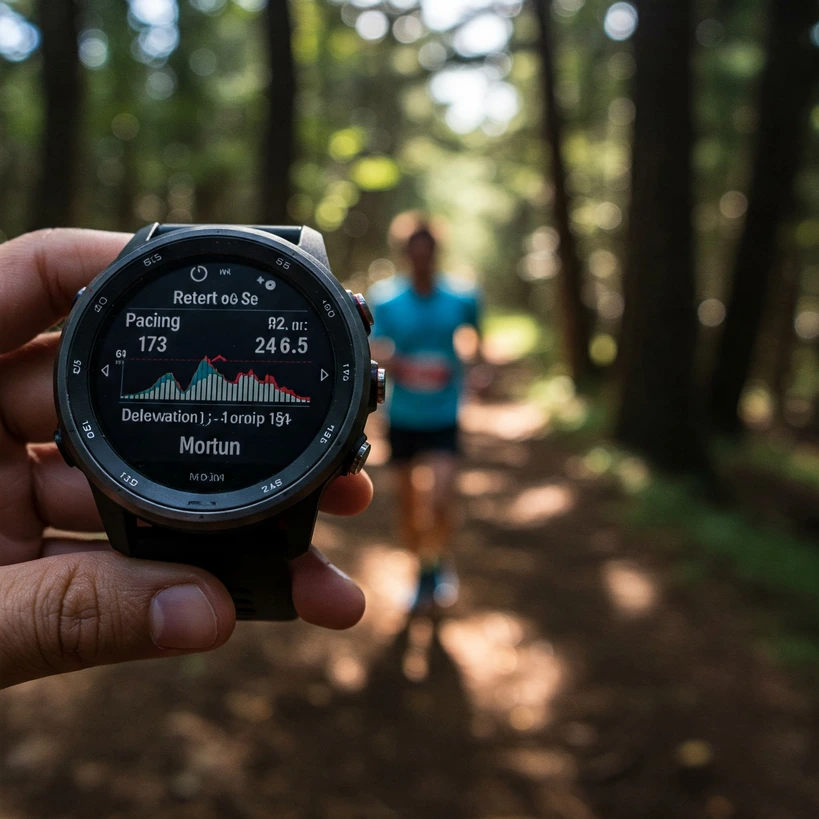
Further Reading & Sources
For those interested in delving deeper into the topics discussed, here are some relevant resources and platforms mentioned:
- How to train for an ultramarathon: The complete guide – Runner’s World
- Physiology and Pathophysiology in Ultra-Marathon Running – PubMed Central (PMC)
- How Will AI Become a Sports Science Tool? – Athletic Lab
- Humango – AI Endurance Training Platform
- TrainAsONE – The AI Running App
- AI Endurance – AI Running, Cycling, and Triathlon Coach
- The role of machine learning methods in physiological explorations of endurance trained athletes – Frontiers
- Ochy – AI running form and gait analysis technology
- Gait Analysis for Runners: What to Expect and How It Can Help – HSS
- Optimizing athletic performance through advanced nutrition strategies: can AI and digital platforms have a role in ultraendurance sports? – PubMed Central (PMC)
- Precision Fuel & Hydration – Sweat Testing and Fueling Strategies
- Diagnostic Applications of AI in Sports: A Comprehensive Review of Injury Risk Prediction Methods – PubMed Central (PMC)
- Machine learning approaches to injury risk prediction in sport – British Journal of Sports Medicine
- How to Use Heart Rate Variability Data in Your Training – HSS
- WHOOP – Health and Fitness Wearable (Mentioned for AI Coach feature)
- Runna – Personalized Training Plans
- Athletica AI – AI-Powered Athletic Training
- How Is AI impacting Sports: Redefining Fair Play and Beyond – Folio3 AI (Discusses Ethics)
Note: Link accessibility and content may change over time. Links accessed April 5, 2025, based on provided research document.
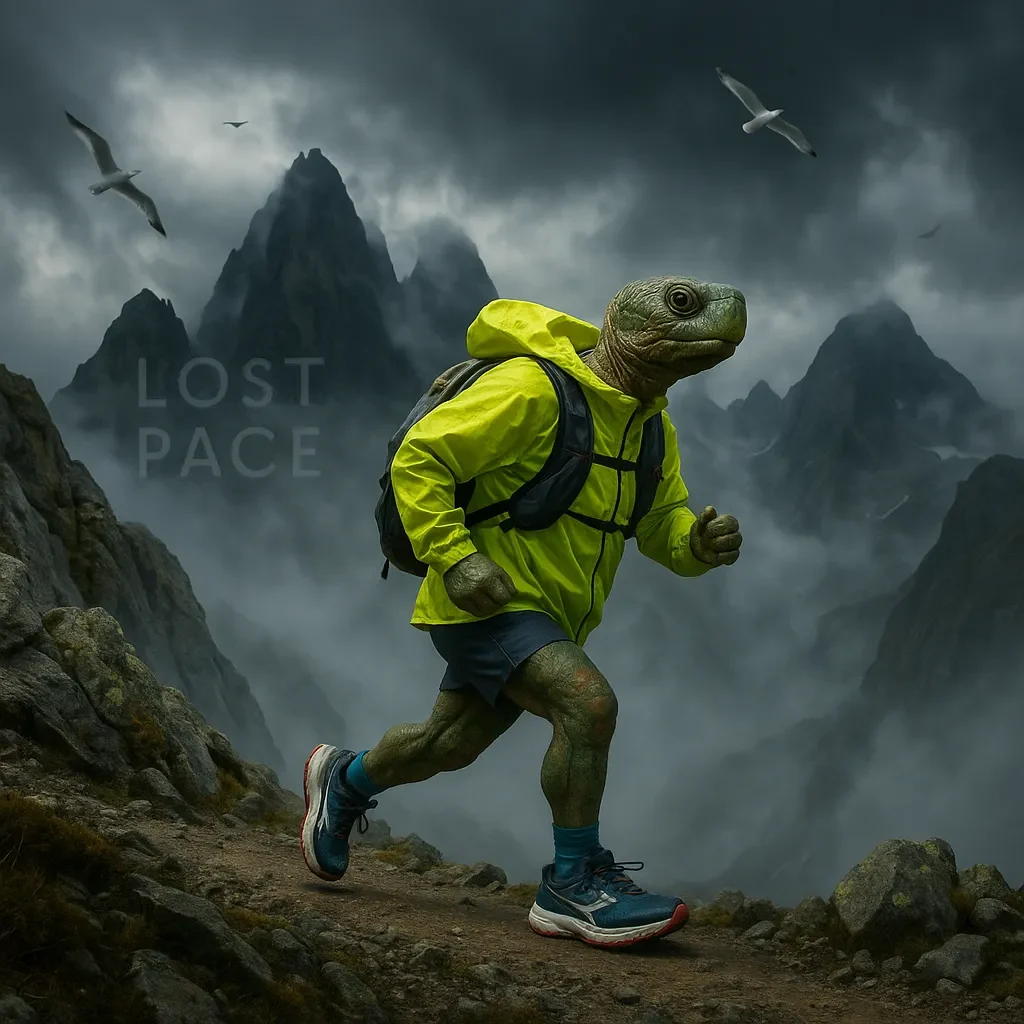
About the Author
Lost Pace is an ultramarathon runner, shoe-tester and the founder of umit.net. Based year-round in Türkiye’s rugged Kaçkar Mountains, he has logged 10,000 + km of technical trail running and completed multiple 50 K–100 K ultras.
Blending mountain grit with data, Lost analyses power (CP 300 W), HRV and nutrition to craft evidence-backed training plans. He has co-written 260 + long-form guides on footwear science, recovery and endurance nutrition, and is a regular beta-tester of AI-driven coaching tools.
When he isn’t chasing PRs or testing midsoles, you’ll find him sharing peer-reviewed research in plain English to help runners train smarter, stay healthier and finish stronger.
Ultrarunner · Data geek · Vegan athlete