Introduction: The Dawn of Intelligent Running
Running, in its purest form, is beautifully simple: one foot in front of the other. Yet, the tools we use to understand and enhance this fundamental human movement have undergone a breathtaking evolution. We’ve journeyed far from the days when performance was measured solely by a ticking stopwatch and progress was judged by the feel of our lungs and legs. The advent of specialized running shoes marked a step forward, followed by the introduction of heart rate monitors that offered a first glimpse into our internal effort. Then came GPS watches, revolutionizing how we tracked distance, pace, and routes, painting a clearer picture of our external performance.
But today, we stand at the threshold of a new era, one defined not just by data collection, but by data intelligence. This transformative shift is powered by the potent synergy of AI and Wearable Technology for Running. These aren’t just incremental improvements; they represent a fundamental reshaping of how we train, race, recover, and understand our bodies as runners. This powerful combination moves beyond simply logging miles; it delves into the why behind our performance, the subtle signals of fatigue, the biomechanical quirks that might lead to injury, and the personalized adjustments needed to unlock our true potential.
Why does this convergence matter so profoundly? Because it democratizes expertise. It brings sophisticated analysis, previously confined to elite sports labs, to the wrists, feet, and pockets of everyday runners. The promise is compelling: training plans that adapt dynamically to your life and your body, real-time coaching cues whispered in your ear mid-run, insights into your unique running form to improve efficiency, and even proactive warnings about potential injuries before they sideline you. It’s about running smarter, safer, and ultimately, achieving goals that once seemed out of reach.
This comprehensive guide will serve as your roadmap through this exciting landscape. We’ll start by defining the core components – AI applications tailored for running and the sophisticated wearable devices that fuel them. We’ll dive deep into the specific ways this technology is revolutionizing the running experience, exploring everything from hyper-personalized training and real-time feedback to intricate gait analysis and injury prevention strategies. We’ll examine the incredible array of data these devices capture and demystify how AI processes this raw information into actionable insights. Crucially, we’ll look at the documented benefits, weigh them against the inherent challenges and valid criticisms – including data accuracy, privacy concerns, and cost. We’ll provide a snapshot of the current market, highlighting key players and products, before gazing into the crystal ball to explore the future trends shaping the road ahead. Join us as we explore the dawn of intelligent running.
Defining the Landscape: Understanding AI and Wearables in the Running Context
To fully grasp the impact of this technological revolution, we first need to clearly define its core components: Artificial Intelligence as applied to running, and the specialized Wearable Technology designed for runners. They are distinct yet deeply interconnected, forming the foundation of the intelligent running experience.
What Exactly is AI in Running Applications?
Artificial Intelligence, in its broader sense, refers to the capability of computer systems to perform tasks that typically require human intelligence. This includes learning from experience, solving problems, understanding language, recognizing patterns, and making decisions. AI isn’t a single entity but rather an umbrella term encompassing various techniques like:
- Machine Learning (ML): Algorithms that allow systems to automatically learn and improve from data without being explicitly programmed. This is the engine behind personalization and prediction in many running apps.
- Deep Learning (DL): A subset of ML using complex neural networks with many layers to analyze vast amounts of data, often excelling at tasks like image recognition (for video gait analysis) or processing complex sensor data over time.
- Natural Language Processing (NLP): Enabling computers to understand and respond to human language, potentially powering AI coaches that can interpret your spoken feedback or answer your training questions.
- Computer Vision: Allowing systems to “see” and interpret images or videos, crucial for apps that analyze running form from smartphone recordings.
In the specific context of running, AI applications are software programs that leverage these techniques to analyze runner-specific data. This data might come directly from wearable sensors, be manually inputted by the user (like perceived effort or race goals), or even gleaned from environmental sources. The primary goal of these AI applications is to move beyond simple data display towards intelligent interpretation and action. They aim to:
- Automate Analysis: Processing complex datasets far quicker and potentially identifying patterns a human might miss.
- Personalize Experiences: Tailoring recommendations, plans, and feedback to the individual runner’s unique physiology, goals, and progress. This includes providing effective AI coaching for runners.
- Enable Informed Decisions: Equipping runners and coaches with insights to make smarter choices about training intensity, recovery, technique adjustments, and injury avoidance.
Think of AI as the “brain” that makes sense of the numbers. It transforms raw data points like heart rate, pace, and cadence into meaningful concepts like “fatigue level,” “optimal training zone,” “gait inefficiency,” or “increased injury risk.” Examples range from dedicated virtual coaching platforms that build entire training schedules to performance analysis tools that dissect every segment of your run.
Defining Wearable Technology for Runners
Wearable technology encompasses any electronic device designed to be worn on the body. This category is broad, including everything from smartwatches and fitness trackers worn like accessories, to sensors integrated into clothing (smart garments), devices implanted in the body, or even temporary electronic tattoos. In the realm of sports, and specifically running, wearables are engineered to capture data relevant to athletic activity and physiological response.
For runners, the most common and impactful wearables include:
- Smartwatches: These are often the central hub, integrating GPS for location tracking, optical heart rate (OHR) sensors, accelerometers and gyroscopes (for motion sensing), barometers (for elevation), and sometimes even SpO2 (blood oxygen) or ECG (electrocardiogram) sensors. They run apps and display data conveniently on the wrist.
- Fitness Bands: Often simpler and more affordable than smartwatches, focusing primarily on activity tracking (steps, distance), heart rate monitoring, and sleep analysis.
- Heart Rate Monitor Chest Straps: These specialized devices use electrical signals (EKG/ECG) to measure heart rate, generally considered more accurate than wrist-based optical sensors, especially during high-intensity intervals or for capturing Heart Rate Variability (HRV).
- GPS Tracking Devices/Pods: Some devices focus solely on providing highly accurate GPS data for pace and distance, sometimes worn on the waist or integrated differently than a watch.
- Motion Sensors / Inertial Measurement Units (IMUs): Often found in foot pods or integrated into watches/insoles, these combine accelerometers, gyroscopes, and sometimes magnetometers to capture detailed biomechanical data like cadence, ground contact time, vertical oscillation, and even calculate running power. These wearable sensors for running analysis provide crucial insights into running form.
- Smart Insoles and Shoes: Embedding pressure sensors or IMUs directly into footwear allows for highly specific data collection about foot strike patterns, pressure distribution, and ground interaction.
- Specialized Sensors: Niche devices are emerging to measure metrics like core body temperature or muscle oxygen saturation, offering deeper physiological insights.
- Smart Garments: Clothing with integrated sensors, such as smart socks that monitor foot landing and cadence.
The primary function of these wearable devices is to act as the data acquisition engine. They are the sensory organs capturing the continuous stream of objective, real-time physiological and biomechanical information generated during a run and throughout the day (like sleep and recovery metrics). This raw data forms the essential input, the fuel, for the AI algorithms to perform their analysis.
The Symbiotic Relationship: Why AI Needs Wearables (and Vice Versa)
It’s crucial to understand that AI and wearable technology for running are not just parallel developments; they are deeply intertwined in a symbiotic relationship. One cannot reach its full potential without the other.
- Wearables Fuel AI: AI algorithms, especially sophisticated machine learning models, thrive on data. Lots of it. Wearable devices provide the rich, continuous, and often objective stream of real-time data that these algorithms need to learn patterns, make predictions, and offer personalized insights. Without wearables, AI running apps would be limited to manually entered data, lacking the granularity and objectivity needed for deep physiological and biomechanical analysis.
- AI Elevates Wearables: Conversely, wearables on their own can sometimes lead to data overload. A runner might see dozens of metrics after a run, but without context or interpretation, this data can be overwhelming or even meaningless. AI provides the crucial “intelligence layer.” It interprets the raw data, identifies significant trends, filters out noise, and translates complex metrics into actionable advice and understandable summaries. AI transforms a passive data logger into an active coach, a diagnostic tool, and a predictive advisor, fundamentally enhancing the value proposition of the wearable device beyond simple tracking.
This interdependence drives innovation in both fields. As sensor technology becomes smaller, more accurate, and capable of measuring new metrics, it unlocks possibilities for more sophisticated AI features. For example, highly accurate HRV data from a chest strap enables more reliable AI-based recovery analysis. Simultaneously, the demand for specific AI-driven insights – like precise injury risk prediction or real-time form correction – pushes manufacturers to develop new and improved wearable sensors for running analysis capable of capturing the necessary data with sufficient fidelity. The development cycles of hardware (wearables) and software (AI applications) are becoming increasingly interconnected, leading to integrated systems where the whole is truly greater than the sum of its parts.
2025 Market Trends: AI & Wearables in Running
2025 is a breakthrough year for AI and wearable technology in running. The market is booming with smarter, more integrated devices and apps, reshaping how runners of all levels train, race, and recover.
- Mass Adoption: Over 45% of recreational runners now use at least one AI-driven app or wearable, a 50% jump since 2023 (Source: Running Industry Association, 2025).
- New Entrants: Major brands like Garmin, Apple, and COROS face fierce competition from emerging startups such as Runna, TrainAsONE, NURVV Run, Ochy, and Runeasi—offering hyper-personalized AI coaching and real-time biomechanical analysis.
- Integration & Ecosystem: The most popular platforms are now multi-device ecosystems, combining smartwatches, foot pods, insoles, and even AI-enabled apparel for a holistic data-driven experience.
- Injury Prevention: AI-driven injury risk scoring and recovery readiness metrics are now standard features on top platforms, and used by 70% of competitive runners.
- Data Privacy: With more granular personal data, 2025 has seen a rise in privacy-focused wearables and apps—GDPR-style transparency and user data control are selling points for European and US brands.
- Future Growth: The global running wearables and AI training market is projected to exceed $6.2 billion USD by the end of 2025 (MarketWatch, Q2 2025).
Product/App | Best For | Main AI Features | Platform | Price (USD) |
---|---|---|---|---|
Garmin Forerunner 965 | All-around, advanced runners | Adaptive training, HRV, race predictions, sleep/coaching | Wearable + App | $599 |
Coros Apex 2 Pro | Ultrarunners, battery life | AI race planning, fatigue tracking, multi-sport | Wearable + App | $449 |
Apple Watch Ultra 2 | iPhone users, smart features | AI coaching, ECG, advanced health metrics, seamless integration | Wearable + App | $799 |
Runna | Personalized training plans | AI-driven adaptive coaching, feedback, weekly adjustment | App | $17/mo |
TrainAsONE | Dynamic, data-driven athletes | Real-time AI plan adjustment, health analytics, advanced injury prediction | App | $12/mo |
NURVV Run | Gait analysis, injury prevention | Real-time footstrike, balance, AI injury risk | Smart insoles + App | $249 |
Ochy | Budget, video-based gait analysis | AI-powered form assessment, feedback | App | $9/mo |
Whoop 4.0 | Recovery, HRV, lifestyle monitoring | AI-based readiness, sleep, daily strain, health trends | Wearable + App | $239 + $30/mo |
AI Running Apps & Wearables: 2025 Face-Off
Choosing the right AI running app or wearable can be overwhelming. Here’s a quick side-by-side comparison of the leading platforms in 2025—so you can match the best tool to your needs, goals, and budget.
Garmin Forerunner 965
👍 Pros: Best for multi-sport, advanced metrics, strong ecosystem👎 Cons: Expensive, learning curve
Apple Watch Ultra 2
👍 Pros: Smart features, health monitoring, stylish👎 Cons: Battery life, not ultra-specific
Runna (App)
👍 Pros: User-friendly, affordable AI coaching👎 Cons: App-only, no hardware
NURVV Run
👍 Pros: Live gait analysis, injury alerts👎 Cons: Needs charging, not for all shoes
TrainAsONE
👍 Pros: Deep AI analytics, device integration👎 Cons: Advanced UI, less beginner-focused
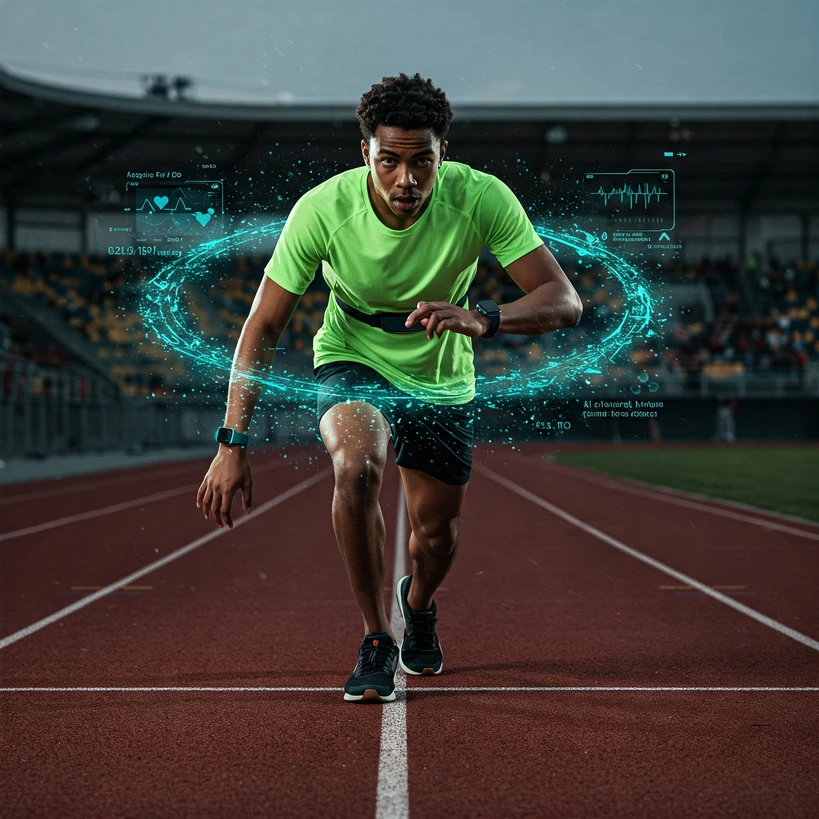
How AI is Revolutionizing Your Run: Key Applications and Benefits
The fusion of AI and wearable technology isn’t just about collecting more data; it’s about fundamentally changing how we run, train, and recover. AI algorithms are being applied in increasingly sophisticated ways to enhance nearly every aspect of the running experience. Let’s delve into the key applications where AI is making the biggest impact.
Personalized and Adaptive Training Plans: Beyond One-Size-Fits-All
Perhaps the most widely adopted application of AI in running is the creation of truly personalized and adaptive training plans. Gone are the days of downloading a static, generic PDF plan designed for an “average” runner targeting a specific race distance. AI coaching for runners takes a radically different, individualized approach.
- Deep Personalization: AI algorithms start by analyzing a vast array of runner-specific data points. This includes:
- Goals: Race distance, target time, general fitness improvement.
- Current Fitness: Assessed through performance history, recent workouts, or even specific fitness tests.
- Performance History: Past race results, training logs, pace zones.
- Physiological Data: Resting heart rate, HRV, sleep quality and duration (often from wearables).
- Biomechanical Data: Insights from gait analysis (if available).
- User Preferences: Preferred running days, long run day, types of workouts enjoyed/disliked.
- Subjective Feedback: How the runner felt during or after previous workouts.
- Contextual Factors: Age, available equipment, time constraints. By synthesizing these factors, AI crafts a training schedule uniquely tailored to the individual runner’s needs, abilities, and life context.
- Dynamic Adaptation: The real magic lies in the adaptive nature of these plans. Unlike static schedules, AI-driven plans are living documents that continuously evolve. The AI monitors progress and adjusts the plan based on:
- Workout Completion: Did you hit the targets for your last run? Was it easier or harder than expected (based on heart rate, pace, perceived effort)?
- Missed Sessions: Life happens. AI can intelligently reschedule or modify upcoming workouts if a session is missed.
- Recovery Status: Using data like HRV, resting heart rate, and sleep scores, AI can detect signs of fatigue or inadequate recovery and proactively adjust training load – perhaps suggesting an easier day or a rest day instead of a hard workout.
- Real-time Feedback: Some systems might even adjust a workout mid-run based on current performance or physiological response.
- User Input: Incorporating the runner’s subjective feedback about how they feel.
- The Advantage: This dynamic, data-driven approach ensures the training load is always optimized – challenging enough to stimulate improvement but not so overwhelming as to lead to burnout or injury. It accounts for the fluctuations in daily life and physiological state, something a static plan simply cannot do. This constant optimization significantly increases the likelihood of consistent training, effective adaptation, and ultimately, achieving one’s running goals. Several popular running apps, such as TrainAsONE, Runna, Humango, and NXT RUN, explicitly leverage AI to deliver this level of personalized and adaptive coaching.
Real-Time Performance Feedback and Coaching: Your AI Coach on the Go
Imagine having a knowledgeable coach running alongside you, offering timely advice and encouragement. This is increasingly becoming a reality thanks to AI-powered real-time feedback delivered through wearables and smartphone apps. By processing data from sensors instantaneously, AI can provide immediate guidance during the run itself.
- Instantaneous Analysis: AI algorithms analyze incoming data streams from GPS (pace, distance), heart rate sensors (effort level), motion sensors (cadence, power), and sometimes even phone cameras (posture).
- Varied Feedback Delivery: This feedback can take multiple forms, often delivered via audio cues through headphones connected to a watch or phone:
- Pacing Guidance: Alerts if you’re running too fast or too slow compared to your target pace for a specific workout segment (e.g., “Slow down, current pace is 7:45/mile, target is 8:00/mile”).
- Effort Management: Warnings if your heart rate exceeds the target zone for the intended workout intensity (e.g., “Heart rate high, ease off slightly”).
- Technique Cues: Suggestions for form correction based on cadence, ground contact time, or other biomechanical metrics (e.g., “Increase cadence,” or potential alerts about foot strike asymmetry from systems like Sensoria Run‘s smart socks).
- Intensity Adjustments: Recommendations to push harder or ease off based on overall workout goals and current physiological response.
- Motivational Prompts: Encouragement during challenging parts of a run or reminders of goals.
- Mimicking the Coach: The goal is to replicate some of the key functions of a human coach during a session – keeping the runner focused, ensuring proper execution of the workout, preventing costly errors in pacing or effort, reinforcing good technique, and providing motivation. Effective AI coaching for runners in real-time can significantly enhance workout quality and adherence. Platforms like Nurvv Run leverage their smart insoles for real-time feedback, while many Garmin and Apple Watch apps offer customizable audio alerts for pace and heart rate zones.
AI-Powered Running Gait Analysis: Understanding Your Biomechanics
How you run – your form or gait – has a significant impact on both performance efficiency and injury risk. Traditionally, detailed gait analysis required expensive laboratory setups with multiple cameras and force plates. AI is now making sophisticated biomechanical insights more accessible to the average runner.
- Democratizing Analysis: AI employs two main techniques for gait analysis outside the lab:
- Computer Vision: Using algorithms to analyze video footage of a runner (often captured via smartphone). The AI identifies body landmarks, tracks joint angles, and calculates various biomechanical parameters frame by frame.
- Inertial Measurement Units (IMUs): Utilizing data from wearable sensors for running analysis, typically placed in foot pods (like Runscribe) or smart insoles (Nurvv Run). These sensors capture detailed motion data (acceleration, rotation) thousands of times per second.
- Key Metrics Uncovered: AI-driven gait analysis can measure and interpret a wide range of metrics, including:
- Temporal Parameters: Cadence (steps per minute), Stride Length, Ground Contact Time (GCT), Flight Time.
- Kinematic Parameters: Vertical Oscillation (how much you bounce), Joint Angles (hip, knee, ankle flexion/extension at different phases), Pelvic Tilt/Rotation.
- Footstrike Pattern: Heel strike, midfoot strike, forefoot strike, degree of pronation/supination.
- Symmetry and Balance: Comparing left vs. right side metrics to identify imbalances.
- Kinetics (often estimated or derived): Impact Forces, Loading Rate (how quickly force is applied upon landing), Running Power.
- Stability: Assessing dynamic stability during the stance phase.
- Actionable Insights: The ultimate goal isn’t just to measure these parameters but to interpret them. AI algorithms compare individual data to normative ranges or ideal patterns (though the validity of universal “ideals” is debated) to:
- Identify potential inefficiencies that waste energy.
- Pinpoint biomechanical flaws often associated with common running injuries (e.g., excessive overpronation, high impact forces, significant asymmetries).
- Provide personalized recommendations, such as specific strengthening exercises, mobility drills, or running form cues, designed to address the identified issues. Apps like Ochy leverage smartphone video analysis, while systems like Runeasi use dedicated sensors for highly detailed 3D analysis often employed by physiotherapists and coaches. This technology empowers runners to understand and refine how they run, potentially leading to greater efficiency and reduced injury likelihood.
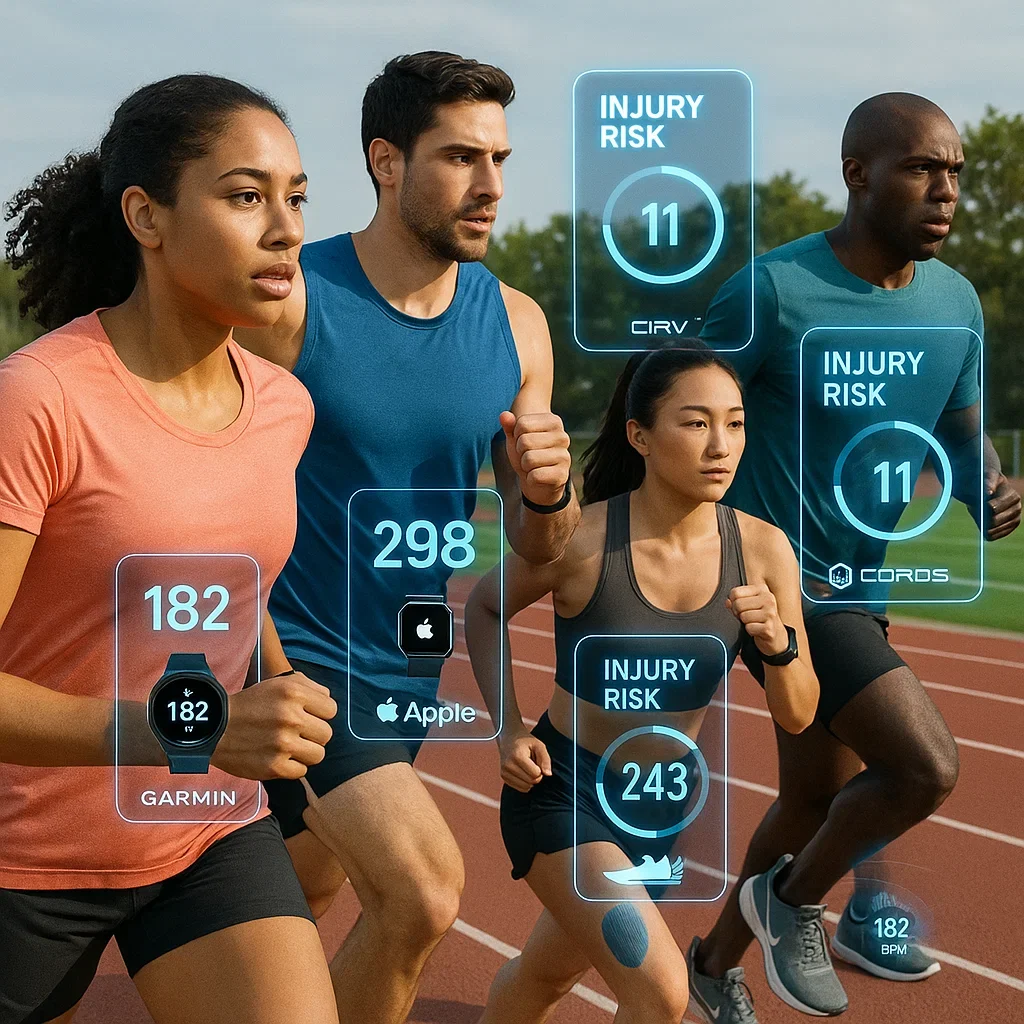
The Holy Grail? AI for Running Injury Prediction and Prevention
Perhaps the most ambitious and potentially impactful application of AI in running is the prediction and prevention of injuries. Running injuries are frustratingly common, often derailing training cycles and diminishing enjoyment of the sport. AI for running injury prevention aims to shift the paradigm from reacting to injuries after they occur to proactively identifying and mitigating risks before pain even begins.
- Proactive vs. Reactive: Traditional approaches often involve managing training load based on general principles or reacting to soreness or pain. AI enables a more proactive stance by looking for subtle warning signs in data.
- Complex Data Integration: Preventing injuries isn’t about a single metric. AI excels at analyzing complex, multi-dimensional datasets by integrating information from various sources:
- Training Load: Monitoring volume, intensity, frequency, and crucially, the rate of change in load (e.g., Acute:Chronic Workload Ratio – ACWR, a key indicator of overload risk).
- Biomechanical Data: Identifying potentially risky movement patterns from gait analysis or continuous monitoring via wearable sensors for running analysis (e.g., increasing asymmetry, high impact transients).
- Physiological Data: Tracking recovery indicators like HRV, resting heart rate, and sleep quality. Sustained low HRV or poor sleep can indicate systemic fatigue and heightened injury susceptibility.
- Historical Data: Incorporating the runner’s past injury history.
- Subjective Data: Potentially including runner-reported feelings of fatigue, soreness, or stress.
- Identifying Risky Patterns: Machine learning algorithms are trained to recognize patterns and correlations within these combined datasets that are known or suspected to precede injuries. This could involve detecting:
- Sudden spikes in training load.
- Gradual negative trends in recovery metrics.
- Emerging biomechanical asymmetries or deviations from the runner’s baseline movement signature.
- Combinations of factors that synergistically increase risk.
- Predictive Alerts and Guidance: Based on the analysis, the AI system can:
- Generate an “injury risk score” or flag potential issues.
- Alert the runner or their coach to heightened risk levels.
- Provide specific, actionable recommendations, such as: reducing training volume/intensity, taking an extra rest day, incorporating specific mobility or strength exercises to address identified weaknesses, or even suggesting seeking professional medical evaluation. While still an evolving field, platforms associated with injury management like PhysiApp or integrated systems like Zepp Coach™ and professional team solutions like Catapult incorporate elements of load management and risk assessment. The ultimate goal of AI for running injury prevention is to keep runners healthier, more consistent, and better able to pursue their goals without interruption.
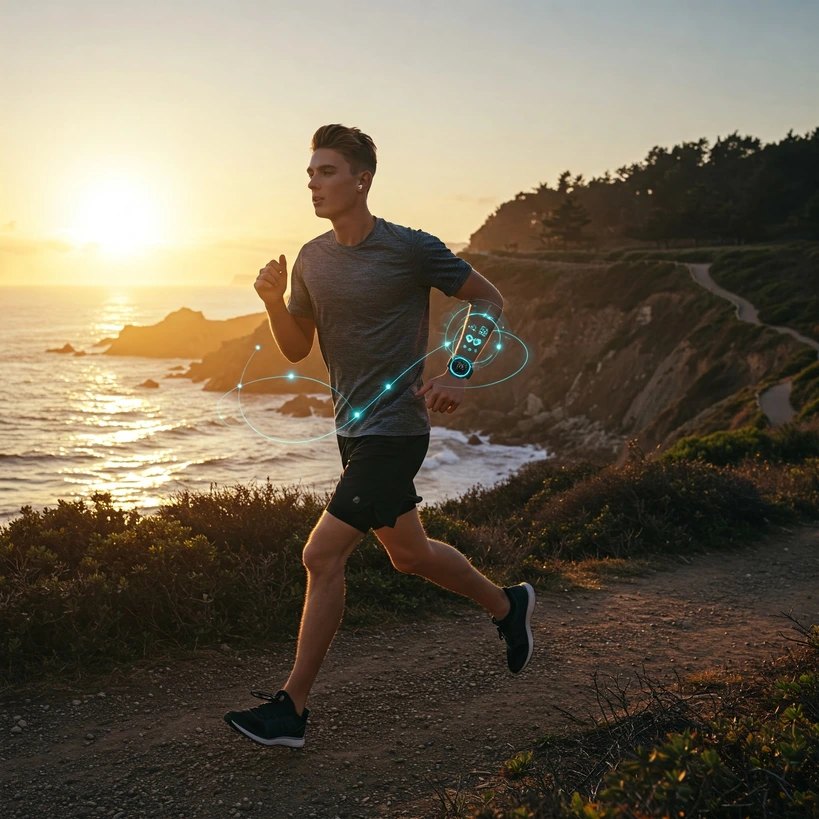
The Data Engine: Wearable Devices Powering AI Insights
The sophisticated analyses performed by AI running applications are entirely dependent on the quality, quantity, and type of data fed into them. Wearable devices are the indispensable “data engines” capturing the raw information about a runner’s body and performance, forming the foundation upon which AI builds its intelligence. Understanding these devices and the data they collect is crucial to appreciating how the entire system works.
Common Types of Wearable Devices for Runners
The market for running wearables is diverse, offering a range of devices with varying capabilities and form factors. Here are the most common types runners encounter:
- Smartwatches: These wrist-worn devices have become the dominant hub for many runners. Leading examples include the Apple Watch series, Garmin‘s extensive Forerunner and Fenix lines, Coros watches popular for their battery life, Suunto devices known for durability, and offerings from Samsung (Galaxy Watch) and Polar. They typically bundle GPS, optical heart rate (OHR) sensors, accelerometers, gyroscopes, and often altimeters. Many also include advanced features like SpO2, ECG, music storage, and smartphone notifications, running complex operating systems that support third-party apps.
- Fitness Bands: Generally simpler and more affordable alternatives to smartwatches, fitness bands (like those from Fitbit, now part of Google) excel at tracking daily activity (steps, calories), basic sleep stages, and continuous heart rate. While less feature-rich for hardcore run tracking than dedicated running watches, they provide valuable baseline health data that can inform AI recovery analysis.
- Heart Rate Monitor Chest Straps: For runners prioritizing heart rate accuracy, especially for Heart Rate Variability (HRV) tracking or high-intensity interval training where wrist-based OHR can falter, chest straps remain the gold standard. Brands like Polar (e.g., H10) and Garmin (e.g., HRM-Pro) use electrocardiography (ECG) sensors to directly measure the heart’s electrical signals, transmitting the data wirelessly to a watch or app.
- GPS Trackers/Pods: While most watches include GPS, some dedicated devices or pods focus solely on providing the most accurate location, pace, and distance data possible, sometimes used in professional team settings (e.g., Catapult systems) or by runners prioritizing absolute GPS precision.
- Motion Sensors / IMUs (Foot Pods): These small devices, often clipped to the shoe (like Stryd, which focuses on running power, or Runscribe, focused on broader biomechanics), contain advanced IMUs. They provide detailed insights into running dynamics that wrist-based sensors often struggle to capture accurately, making them valuable wearable sensors for running analysis focused on form.
- Smart Insoles and Shoes: Taking sensor integration a step further, these embed sensors directly within the shoe’s insole. Nurvv Run utilizes insoles with numerous pressure sensors alongside IMUs to map foot pressure distribution and capture detailed gait metrics. Some shoe manufacturers like Under Armour have also experimented with embedded chips that track run data.
- Specialized Sensors: A growing category includes niche devices targeting specific physiological metrics. Examples include sensors measuring core body temperature (important for heat acclimatization and safety) or muscle oxygen saturation (potentially indicating muscle fatigue or performance limits).
- Smart Garments: This category involves weaving sensors directly into clothing. Sensoria‘s smart socks, which monitor cadence and foot landing patterns, are a prime example relevant to runners. While less common than watches or pods, the potential for integrating sensors seamlessly into apparel is significant.
- Emerging Form Factors: Technologies like Smart Rings (e.g., Oura Ring) are gaining traction, offering a different way to continuously monitor metrics like sleep, HRV, and temperature, providing valuable recovery data for AI analysis without requiring a wrist-worn device 24/7.
Key Biometric and Performance Data Collected by Wearables
The array of data points collected by this diverse range of wearables is vast. AI algorithms synthesize these inputs to build a comprehensive picture of the runner. Key data categories include:
- Motion / Performance Data:
- Pace / Speed: How fast you are running (usually from GPS, sometimes estimated by accelerometers).
- Distance: How far you have run (primarily GPS).
- Cadence: Steps per minute (from accelerometers/IMUs).
- Stride Length: Distance covered per stride (often calculated from pace and cadence).
- Ground Contact Time (GCT): How long each foot stays on the ground (from foot pods/IMUs).
- Vertical Oscillation: How much your torso bounces up and down with each stride (from accelerometers/IMUs).
- Running Power: An estimate of the mechanical power output during running (calculated using pace, elevation change, sometimes wind, and body weight; measured more directly by devices like Stryd).
- Acceleration / Deceleration: Rates of change in speed (from IMUs).
- Positional Data / Heatmaps: GPS track of your run.
- Physiological Data:
- Heart Rate (HR): Beats per minute, indicating cardiovascular effort (from OHR or ECG chest straps).
- Heart Rate Variability (HRV): The variation in time between heartbeats, a key indicator of autonomic nervous system balance and recovery status (best measured by ECG, though OHR algorithms are improving).
- Calories Burned: An estimation based on HR, duration, intensity, and personal metrics.
- Sleep: Duration, stages (light, deep, REM), quality scores, interruptions (from accelerometers, OHR).
- Respiration Rate: Breaths per minute (often derived from HR data or chest strap movements).
- Blood Oxygen Saturation (SpO2): Percentage of oxygenated hemoglobin in the blood (via specific OHR sensors, often used for altitude acclimatization assessment or sleep monitoring).
- Skin Temperature: Measured at the surface (wrist, finger).
- Core Body Temperature: Internal body temperature (requires specialized sensors).
- ECG: Electrical trace of the heart (available on some high-end watches, primarily for atrial fibrillation detection but also provides raw data for accurate HRV).
- Muscle Fatigue / Activity: Potential future metrics from EMG or NIRS sensors.
- Hydration Levels: Currently estimated or requires specialized patches; an area of active research.
- Stress Levels: Often derived from HRV analysis and other physiological markers.
- Biomechanical Data (often requires specialized sensors/gait analysis):
- Footstrike Pattern: Where the foot initially contacts the ground (heel, midfoot, forefoot).
- Pronation / Supination: The inward/outward rolling motion of the foot during stance.
- Joint Angles: Kinematic measurements of hip, knee, ankle movement (typically via video analysis or advanced IMU systems).
- Symmetry / Balance: Comparing metrics between the left and right sides of the body.
- Impact Forces / Loading Rate: Measuring the forces experienced upon landing and how quickly they are applied (from pressure insoles or advanced IMUs).
- Pressure Distribution: Mapping how pressure is distributed across the sole of the foot during stance (from smart insoles).
Here’s a summary table illustrating which devices typically collect which primary data types:
Device Type | Primary Data Collected |
---|---|
Smartwatch | HR (OHR), HRV (estimated), Pace, Distance, Cadence, Calories, Sleep, SpO2, GPS Location, (some: EKG, Barometric Altitude, Running Power estimated) |
Fitness Band | HR (OHR), Steps, Distance (estimated), Calories, Sleep |
HR Chest Strap | HR (ECG-based), HRV (high accuracy) |
GPS Tracker/Pod | Pace, Distance, Location (high accuracy GPS) |
Foot Pod / IMU Sensor | Cadence, Ground Contact Time, Vertical Oscillation, Running Power, Stride Length, Footstrike (derived), Impact Forces (some), Symmetry |
Smart Insole | Pressure Distribution, Footstrike, Ground Contact Time, Cadence, (some: IMU data like pronation, impact) |
Core Temp Sensor | Core Body Temperature |
Smart Socks (e.g. Sensoria) | Cadence, Foot Landing Pattern |
Smart Ring (e.g. Oura) | Sleep Stages, HRV, Temperature, Activity Level, Readiness Score |
Why Specialized Sensors Still Matter: Accuracy vs. Convenience
While the convenience of having multiple sensors packed into a single smartwatch is undeniable, there’s often a trade-off between convenience and accuracy for certain metrics. Specialized sensors frequently provide higher fidelity data that is critical for reliable AI analysis, particularly for more sensitive applications:
- HRV Accuracy: While optical sensors on watches are improving, ECG-based chest straps remain significantly more accurate for capturing the beat-to-beat variations needed for precise HRV analysis, a cornerstone of AI-driven recovery and readiness scores.
- Biomechanical Detail: Wrist-based sensors can estimate cadence reasonably well, but they struggle to capture nuanced metrics like ground contact time, vertical oscillation, footstrike pattern, or impact forces. Foot pods or smart insoles, being closer to the action, provide far more direct and reliable data for detailed gait analysis using wearable sensors for running analysis.
- Running Power: While some watches estimate power, dedicated devices like Stryd use specific algorithms and direct measurements (factoring in wind) to provide a more consistent and potentially actionable power metric.
- Temperature: Skin temperature measured at the wrist can be influenced by ambient conditions. Dedicated core body temperature sensors provide a more physiologically relevant metric for assessing heat stress and adaptation.
The implication is that AI algorithms perform best with high-quality input data. For runners seeking the most accurate AI-driven insights, especially concerning detailed form analysis or precise physiological monitoring (like recovery status or injury risk factors), relying solely on a smartwatch might not be sufficient. Often, a multi-device ecosystem – perhaps combining a GPS watch with a chest strap and/or foot pods – is necessary to provide the AI with the comprehensive, high-fidelity data it needs to perform optimally. This, of course, leads to considerations of cost and potential integration challenges between different devices and platforms.
From Raw Data to Actionable Insights: The AI Processing Pipeline
Collecting vast amounts of data from wearable sensors is only the first step. The real value emerges when Artificial Intelligence processes this raw information, transforming seemingly chaotic streams of numbers into understandable, meaningful, and actionable insights for the runner. This processing pipeline typically involves several key stages.
How AI Algorithms Analyze Wearable Data
The journey from a sensor reading to a personalized recommendation involves sophisticated computational steps:
- Data Pre-processing: Raw sensor data is rarely perfect. It can be noisy (e.g., inaccurate HR spikes due to sensor movement), contain gaps (e.g., temporary GPS signal loss), or be inconsistent across different devices or sessions. The first crucial step involves cleaning and preparing the data. This includes:
- Noise Filtering: Applying algorithms to smooth out erratic readings.
- Outlier Removal: Identifying and handling data points that are statistically improbable.
- Handling Missing Values: Using techniques like interpolation or imputation to fill gaps logically.
- Normalization/Standardization: Scaling data to a common range so that different metrics can be compared fairly (e.g., ensuring pace and heart rate contribute appropriately to an effort score).
- Aggregation: Summarizing data over specific periods (e.g., calculating average heart rate during an interval, total distance for a run). This stage ensures the data is clean, consistent, and in a suitable format for the subsequent analysis steps.
- Feature Extraction: Raw sensor signals (like the waveform from an optical heart rate sensor or the continuous acceleration data from an IMU) often aren’t directly interpretable. Feature extraction involves identifying and calculating meaningful characteristics or “features” from these signals. Examples include:
- Calculating various HRV metrics (like SDNN or RMSSD) from beat-to-beat interval data.
- Identifying distinct phases of the running gait cycle (stance, swing) from IMU data.
- Computing stride parameters (GCT, vertical oscillation, cadence) from accelerometer signals.
- Quantifying specific movement patterns relevant to form analysis. This step reduces the dimensionality of the data while highlighting the aspects most relevant to running performance and physiology.
- Pattern Recognition and Learning: This is where the core Machine Learning (ML) and Deep Learning (DL) algorithms come into play. Using the cleaned and feature-extracted data, these algorithms are trained to:
- Identify Patterns: Recognizing recurring relationships or sequences in the data (e.g., how heart rate typically responds to changes in pace for a specific runner).
- Detect Trends: Identifying gradual changes over time (e.g., a slow decline in HRV indicating accumulating fatigue, an improvement in GCT suggesting better form).
- Find Correlations: Understanding how different metrics relate to each other (e.g., the correlation between cadence and vertical oscillation).
- Spot Anomalies: Flagging data points or patterns that deviate significantly from the runner’s established baseline or expected norms (which could indicate overtraining, potential injury, or sensor malfunction). A wide range of models might be used, from simpler regression or classification algorithms (like Random Forests, Support Vector Machines) to more complex neural networks (ANNs, CNNs for spatial data like pressure maps, RNNs/LSTMs for time-series data like HR over a run).
- Contextualization: A single data point rarely tells the whole story. AI excels at considering multiple data streams simultaneously to provide contextually relevant analysis. For instance, a high heart rate reading is expected during a hard interval session but would be concerning during rest. AI considers:
- The type of workout being performed.
- The runner’s historical data and personal baselines.
- Recent training load and recovery status.
- Environmental factors (temperature, humidity, altitude – if available).
- User-provided subjective feedback. By integrating context, AI can provide much more nuanced and accurate interpretations.
- Prediction and Forecasting: Based on the patterns learned and the current context, AI models can be used to make predictions about future outcomes. This includes:
- Estimating race performance potential based on current fitness.
- Forecasting fatigue levels for upcoming days.
- Assessing the probability of injury risk based on current load, biomechanics, and recovery.
- Predicting the physiological response to planned future workouts.
Transforming Complex Data into Runner-Friendly Analytics and Recommendations
The ultimate goal of this complex processing pipeline is to translate the intricate analysis into information that the runner can easily understand and act upon. AI achieves this through several outputs:
- Synthesized Performance & Recovery Metrics: Instead of presenting dozens of raw data points, AI often calculates summary scores or indices. Examples include:
- Training Load Scores: Quantifying the physiological stress of workouts (e.g., Garmin’s Training Load, Strava’s Relative Effort, Catapult’s PlayerLoad™).
- Fitness Level Estimates: Such as VO2 Max estimations derived from pace and heart rate data during runs.
- Recovery Scores / Readiness Metrics: Combining HRV, sleep data, and recent load to provide a simple score indicating preparedness for training (e.g., Whoop Recovery, Garmin Body Battery™/Training Readiness).
- Personalized Insights & Feedback: AI delivers specific, contextualized observations based on the analysis. These insights go beyond just stating the numbers:
- “Your HRV dropped significantly after yesterday’s hard workout, suggesting you need more recovery today.”
- “Your ground contact time was shorter on average during this run compared to last week, indicating improved efficiency.”
- “Your cadence consistently decreased during the last mile of your long run; focus on maintaining quick steps when fatigued.”
- Actionable Recommendations: The most valuable output is often direct guidance on what the runner should do:
- “Consider replacing tomorrow’s planned speed session with an easy run due to your low recovery score.”
- “Focus on incorporating drills to improve hip extension based on your gait analysis.”
- “Your acute:chronic workload ratio is high; reduce intensity this week to lower injury risk.”
- “Based on your recent performance and recovery trends, you are ready to increase your long run distance.”
- User-Friendly Visualization: Presenting complex data and insights in intuitive formats within the companion app is crucial. This includes:
- Graphs showing trends over time (e.g., HRV, resting HR, training load).
- Charts comparing different runs or workout segments.
- Dashboards summarizing key daily or weekly metrics.
- Visual representations of gait mechanics or pressure distribution.
Peeking Inside the “Black Box”: The Challenge of Explainable AI (XAI)
While AI can perform incredibly complex analyses, a significant challenge, particularly with advanced deep learning models, is their “black box” nature. It can sometimes be difficult, even for the developers, to understand precisely why the AI arrived at a specific conclusion or recommendation.
- Lack of Transparency: Complex neural networks learn intricate, non-linear relationships within data, but they may not provide a simple, step-by-step explanation for their reasoning.
- Impact on Trust and Adoption: This opacity can be a barrier to trust. If an AI recommendation contradicts a coach’s experience or a runner’s intuition, the lack of a clear explanation makes it hard to accept the advice confidently. Users might wonder: Was it based on solid evidence, or a glitch in the algorithm?
- The Need for Explainable AI (XAI): Recognizing this limitation, there’s a growing focus in the AI field on developing XAI techniques. The goal is to create models that are not only accurate but also provide clear, understandable justifications for their outputs. This could involve highlighting the key data points that influenced a decision or providing simpler, rule-based approximations of the complex model’s logic. Building more transparent and interpretable AI is crucial for fostering better human-AI collaboration and increasing user confidence in these powerful tools.
The Learning Loop: How AI Gets Smarter Over Time
A key characteristic of many AI systems, particularly those using Machine Learning, is their ability to learn and improve over time. The more data they process, the better they become at understanding patterns and making accurate predictions.
- Data-Driven Refinement: As a runner consistently uses an AI-powered app and its associated wearables, they feed the system more data – more workout results, more physiological responses (HR, HRV), more sleep patterns, and potentially more subjective feedback.
- Enhanced Personalization: With this growing dataset specific to the individual, the AI model can refine its understanding of that runner’s unique physiology, training responses, recovery patterns, and preferences. Initial recommendations might be based on broader population data, but over time, they become increasingly tailored to the individual “n=1”.
- Increasing Value: This continuous learning loop means that the value of an AI running application can actually increase the longer and more consistently it’s used. The insights become more accurate, the predictions more reliable, and the recommendations more relevant.
- The “Cold Start” Problem: The flip side of this is the “cold start” issue. When a new user starts with an AI app, the system initially has limited data, so the early insights and recommendations might be less personalized or accurate until enough data has been collected to build a reliable individual profile. This highlights the importance of consistent usage, especially during the initial phases, to maximize the benefits of the AI’s learning capabilities.
Measuring the Impact: Documented Benefits (and Caveats) of AI in Running
The proliferation of AI-powered running apps and wearables is driven by the promise of tangible benefits for runners. From smashing personal bests to staying injury-free, the potential impact is significant. Let’s examine the key areas where these technologies are claimed to make a difference, while also acknowledging the nuances in the supporting evidence.
Enhancing Running Performance: Faster, Further, More Efficiently
One of the primary draws of AI running tech is its potential to boost performance. This is achieved through several mechanisms:
- Optimized Training Stimulus: By delivering personalized and adaptive training plans, AI aims to ensure that every workout provides the right stimulus at the right time. It helps runners avoid the pitfalls of undertraining (not improving) or overtraining (leading to fatigue or injury), thereby maximizing physiological adaptation and fitness gains. The constant adjustments based on recovery data (like HRV and sleep) ensure that hard workouts are performed when the body is ready to benefit most. This intelligent modulation of training stress is key to long-term progress.
- Improved Running Economy: Efficiency matters. Running faster isn’t just about cardiovascular fitness; it’s also about minimizing wasted energy. AI-powered gait analysis tools can identify biomechanical inefficiencies – like excessive vertical oscillation, overly long ground contact times, or poor posture – that increase the energy cost of running. By providing targeted feedback and corrective exercise recommendations, these tools aim to help runners refine their form, potentially leading to significant improvements in running economy (using less energy at the same pace). Some sources suggest athletes using specific gait analysis software have seen notable improvements in efficiency and speed, though independent verification of such claims is often needed.
- Smarter Pacing and Effort Management: Real-time feedback during runs helps runners execute workouts more effectively. AI coaches can provide cues to maintain target paces during intervals, prevent going out too fast in races or long runs, and manage effort based on heart rate zones, ensuring the intended physiological system is being trained. This leads to higher quality training sessions and potentially better race execution.
- Anecdotal Success: Many users of AI coaching apps report achieving new personal bests (PBs) across various distances, attributing their success to the structured, personalized, and adaptive nature of the training prescribed by the AI. While anecdotal, these widespread reports suggest a positive impact for many runners. AI coaching for runners is frequently cited as a key factor in these successes.
Boosting Motivation and Engagement: Staying Consistent and Inspired
Consistency is arguably the most critical factor in running improvement. However, maintaining motivation day after day can be challenging. AI and wearable technology incorporate various features designed to keep runners engaged and committed to their training:
- Personalization and Progress Tracking: Feeling that a plan is truly yours and seeing measurable progress are powerful motivators. AI delivers personalized schedules, and wearables meticulously track workouts, providing visual feedback on improvements in pace, distance, fitness scores (like VO2 Max estimates), and consistency streaks.
- Goal Setting and Achievement: AI platforms often help users set realistic yet challenging goals (e.g., completing a marathon, hitting a specific race time) and structure the training to reach them. Achieving intermediate milestones and receiving positive feedback reinforces commitment.
- Real-Time Feedback and Virtual Coaching: The interactive nature of real-time coaching cues can make solo runs feel less monotonous and more purposeful. Having an “AI coach” providing guidance and encouragement can enhance engagement during the activity itself.
- Gamification Elements: Many platforms incorporate game-like features such as earning badges for achievements (e.g., longest run, fastest 5k), completing challenges, reaching milestones, or comparing progress with friends on social leaderboards (like Strava segments). These elements add an element of fun and friendly competition.
- Novelty and Data Insights: For many runners, the technology itself is engaging. Exploring the wealth of data collected, understanding trends, and learning more about their own bodies can be intrinsically motivating. Some studies and surveys indicate that users of wearable technology report increased motivation levels, often citing the real-time feedback and tracking capabilities as key factors. Higher engagement rates have also been reported for users of AI-based fitness platforms compared to traditional methods.
Preventing Injuries and Supporting Recovery: Running Smarter, Not Just Harder
Staying healthy is paramount for consistent training and long-term enjoyment of running. AI for running injury prevention and recovery monitoring represents a significant area of focus:
- Intelligent Load Management: AI algorithms can track training load using sophisticated metrics like the Acute:Chronic Workload Ratio (ACWR), which compares recent (acute) load to longer-term (chronic) load. This helps identify potentially dangerous spikes in training that dramatically increase injury risk. AI can provide warnings or automatically adjust plans to ensure load progresses gradually and safely.
- Biomechanical Risk Factor Identification: Gait analysis tools can pinpoint movement patterns associated with common running injuries (e.g., excessive impact forces, significant asymmetry, overpronation). AI can flag these risks and suggest corrective exercises or form adjustments. Real-time feedback systems might even provide immediate cues during a run if risky movements are detected.
- Objective Recovery Monitoring: AI leverages wearable data – particularly Heart Rate Variability (HRV), resting heart rate, and sleep quality/quantity – to provide objective insights into the body’s recovery status and readiness for training. Instead of relying solely on subjective feeling, runners get data-driven guidance on whether to push hard, take it easy, or prioritize rest.
- Proactive Adjustments: By integrating load management, biomechanical analysis, and recovery monitoring, AI can proactively suggest modifications to training before overtraining or excessive strain leads to injury. This might involve swapping a hard workout for an easy one, incorporating extra rest days, or reducing overall volume. User anecdotes often mention completing training cycles injury-free while using AI coaching platforms. AI tools can also potentially guide the rehabilitation process after an injury occurs.
A Note on Evidence: Correlation vs. Causation
While the potential benefits are compelling and widely reported by users and developers, it’s crucial to approach the evidence with a critical eye:
- The Causation Challenge: It’s difficult to definitively prove that the AI caused the performance improvement or injury prevention. Runners who invest in and consistently use advanced technology might already be more motivated, disciplined, or knowledgeable than average. Is it the AI itself, or the type of person who uses it, that leads to better outcomes? This correlation vs. causation dilemma is hard to untangle without rigorous experimental design.
- Quality of Evidence: Much of the supporting evidence comes from company marketing materials, user testimonials, or studies that may not be peer-reviewed, lack control groups, or have small sample sizes. While valuable, this doesn’t meet the gold standard of scientific proof. Rigorous, independent, large-scale randomized controlled trials specifically investigating the efficacy of AI running applications are still relatively scarce. Some studies have even shown mixed results or potential downsides, such as a decrease in autonomous motivation over time with certain fitness trackers.
- Cautious Interpretation Required: Therefore, while we can discuss the documented and potential benefits based on available information and logical mechanisms, it’s important to acknowledge the limitations of the current evidence base. We should differentiate between features claimed by apps, anecdotal reports, and findings validated by robust scientific research. More independent investigation is needed to fully quantify the true impact of these technologies.
The Interconnectedness of Benefits
It’s also important to recognize that these benefits are often interconnected, creating a potential positive feedback loop:
- Performance -> Motivation: Achieving performance goals (running faster, completing a race) is highly motivating, encouraging continued engagement with the training process and the tools facilitating it.
- Health -> Consistency -> Performance: Staying injury-free allows for uninterrupted, consistent training, which is the bedrock of performance improvement. Consistency also reinforces motivation.
- Engagement -> Data -> Better AI -> Benefits: Consistent use of the technology provides more data for the AI, leading to better personalization and more accurate insights, which in turn enhance performance, motivation, and injury prevention efforts.
Conversely, negative experiences in one area can impact others. Inaccurate data leading to poor training advice could hurt performance, decrease motivation, and even increase injury risk. Therefore, evaluating these technologies requires a holistic view, considering their overall impact on the runner’s journey rather than focusing solely on isolated metrics or features. A system might excel at predicting injury risk but fail if it’s demotivating or provides flawed performance feedback.
Navigating the Hurdles: Challenges and Criticisms of AI and Wearable Tech
Despite the exciting potential and growing popularity of AI and wearable technology in running, it’s essential to acknowledge the significant challenges and criticisms associated with these tools. These hurdles can limit their effectiveness, raise ethical concerns, and impact user trust and adoption.
Data Accuracy, Reliability, and Algorithmic Bias: The “Garbage In, Garbage Out” Problem
The entire premise of AI-driven insights rests on the quality of the input data. Unfortunately, data from wearable sensors is not always perfectly accurate or reliable.
- Sensor Limitations:
- GPS: Accuracy can be affected by environmental factors like tall buildings, dense tree cover, tunnels, or even weather conditions, leading to errors in pace and distance measurement. Different devices also have varying GPS chipset quality.
- Optical Heart Rate (OHR): Wrist-based sensors can struggle during high-intensity intervals (due to rapid HR changes and motion), in cold weather (vasoconstriction), or with individuals who have darker skin tones or tattoos, leading to inaccurate readings. Motion artifacts (the sensor moving on the skin) are a common issue.
- Accelerometers/IMUs: While great for detecting motion, deriving precise biomechanical metrics like ground contact time or vertical oscillation from the wrist can be less accurate than from sensors on the foot or torso. Calibration and placement are critical.
- Consequences of Inaccurate Data: If the sensors feed the AI flawed data, the resulting analysis, insights, predictions, and recommendations will also be flawed. This is the classic “garbage in, garbage out” principle. Inaccurate data could lead to:
- Incorrect training zone calculations, resulting in workouts that are too hard or too easy.
- Flawed assessment of fitness levels or recovery status.
- Unnecessary anxiety due to false positive alerts (e.g., detecting a heart rate spike that wasn’t real).
- False reassurance by missing genuine warning signs (e.g., underestimating training load due to inaccurate pace).
- Misleading gait analysis feedback.
- Validation Challenges: Rigorously validating the accuracy of consumer wearables against gold-standard laboratory equipment is complex and often not thoroughly performed or transparently reported by manufacturers. Accuracy can vary significantly between devices, metrics, and conditions.
- Algorithmic Bias: AI models are trained on data. If the training data is not diverse or representative of all users, the resulting algorithm can exhibit bias. For example, an OHR algorithm trained predominantly on lighter skin tones might perform poorly for users with darker skin. Similarly, gait analysis models might be based on normative data derived from specific populations, which may not be universally applicable, potentially leading to flawed assessments for runners outside that demographic.
Privacy, Security, and Ethical Considerations: Protecting Sensitive Data
Wearable devices and their companion apps collect an unprecedented amount of highly personal and sensitive health data – where you run, how fast, your heart rate patterns, sleep quality, weight, and potentially much more. This data concentration creates significant privacy and security risks.
- Data Sensitivity: This isn’t just step data; it’s intimate health information that users rightfully expect to be protected.
- Security Risks: Centralized repositories of health data are attractive targets for hackers. Data breaches can expose highly personal information. Weak security practices in apps or devices can create vulnerabilities.
- Privacy Concerns:
- Unauthorized Sharing: There are concerns about data being shared with or sold to third parties (advertisers, data brokers, potentially even insurance companies) without explicit, informed consent. Privacy policies can be opaque or change over time.
- Lack of Transparency: Users often lack clarity on exactly what data is being collected, how it’s being used, who it’s being shared with, and how long it’s being stored.
- Potential for Misuse: Concerns exist that collected health data could potentially be used in ways detrimental to the user, such as influencing insurance premiums or even being used in legal contexts. The aggregation of location and health data can create detailed user profiles.
- Data Ownership and Control: Who actually owns the data generated by the user? Users often lack meaningful control over their own data, including the ability to easily delete it permanently from all company servers.
- Regulatory Compliance: Adherence to data protection regulations like Europe’s GDPR (General Data Protection Regulation) or health-specific laws like HIPAA (in the US, if data is used in a healthcare context) is crucial. These regulations mandate principles like explicit consent for processing sensitive health data, data minimization (collecting only necessary data), purpose limitation, transparency, security measures, and user rights (access, correction, deletion). Ensuring compliance, especially with cross-border data transfers, is complex.
- Ethical Balance: Beyond legal compliance, there are ethical considerations. Companies need to balance the utility derived from data analysis with the fundamental right to individual privacy. There’s a need to avoid creating systems that feel overly intrusive, manipulative, or promote unhealthy surveillance. Fairness in how data is used and how algorithms affect different users is also a key ethical concern.
Cost, Accessibility, and the Digital Divide: Is Smart Running Only for the Few?
While the technology offers exciting possibilities, accessing the best tools often comes at a significant cost.
- Financial Barriers: High-end GPS watches, specialized sensors (foot pods, core temp sensors), smart insoles, and premium subscription fees for AI coaching apps can add up quickly, making the most advanced features inaccessible to many runners. Some devices like Nurvv Run have launched with price tags in the hundreds of dollars, plus potential ongoing costs.
- Equity and Health Disparities: This cost barrier can exacerbate existing health inequalities. If the benefits of improved performance, health monitoring, and injury prevention are disproportionately available to wealthier individuals, it could widen the gap in health outcomes and athletic opportunities. Studies have shown that adoption rates for activity trackers can be lower among lower-income groups.
- Other Access Barriers: Effective use of these technologies typically requires owning a compatible smartphone and possessing a certain level of digital literacy to navigate the apps, interpret the data, and manage the devices. This can exclude individuals who lack access to modern smartphones or are less comfortable with technology.
The Risk of Over-Reliance and Skill Atrophy: Trusting Tech Too Much?
While AI and data can provide valuable insights, there’s a potential downside to becoming overly reliant on technology.
- Ignoring Bodily Intuition: Runners might start to distrust or ignore their own body’s signals (fatigue, pain, perceived effort) in favor of what the watch or app tells them. This can lead to pushing through genuine warning signs if the data looks “good,” or unnecessary anxiety if the data looks “bad” despite feeling fine. Learning to listen to one’s body is a crucial skill for any runner.
- Misinterpretation and False Security: Complex data requires careful interpretation. Users might misunderstand metrics or place too much faith in potentially inaccurate outputs, leading to poor decisions (e.g., overtraining based on an inflated VO2 Max estimate) or a false sense of security regarding injury risk.
- Deskilling of Coaches and Athletes: Coaches might become overly dependent on data dashboards, potentially diminishing their observational skills or the importance of direct athlete communication and subjective feedback. Athletes might lose the ability to self-regulate pace and effort effectively without constant technological guidance.
- Finding the Balance: The key is to use technology as a tool to augment, not replace, human judgment, intuition, and experience. Data should inform decisions, not dictate them blindly. Maintaining a connection with one’s own body and fostering strong coach-athlete communication remains essential.
Technical and User Experience Limitations: Everyday Frustrations
Beyond the conceptual challenges, practical limitations can also hinder the user experience:
- Hardware Constraints: Smartwatch screens are small, limiting the amount of data that can be displayed effectively during a run. Battery life remains a significant constraint, especially for longer endurance events (ultramarathons) or multi-day use without charging. Device durability can also be a concern.
- Software and Integration Issues:
- Interoperability: Getting different devices and platforms (e.g., a Garmin watch, a Whoop strap, and a specific AI coaching app) to sync data seamlessly can sometimes be challenging due to incompatible ecosystems or data standards.
- App Complexity: Some apps can be overly complex or unintuitive, presenting a steep learning curve for users.
- Connectivity: Reliable Bluetooth connections between devices and phones are crucial but can sometimes be finicky. Syncing data requires internet access.
- Updates: Frequent software updates are necessary for bug fixes and new features but can sometimes introduce new problems or require re-learning interfaces.
The Interplay of Challenges and the Trust Deficit
Crucially, many of these challenges are interconnected and can contribute to an overall trust deficit:
- Trade-offs: Improving sensor accuracy often requires more sophisticated (and expensive) hardware. Adding more complex AI features can increase battery consumption and app complexity. Stricter privacy measures might limit the amount or type of data available for training more powerful AI models. Development involves constantly balancing functionality, accuracy, cost, usability, and privacy.
- Erosion of Trust: When users experience inaccurate data (Challenge 7.1), worry about how their data is being used (Challenge 7.2), or find the AI’s recommendations opaque and unexplainable (the “Black Box” problem discussed earlier), their trust in the technology diminishes.
- Impact on Adoption and Use: Even if a technology has the potential for significant benefits, a lack of user trust can prevent its adoption or lead to users ignoring its recommendations, rendering it ineffective. If a runner doesn’t trust the recovery score, they won’t adjust their training based on it.
Building and maintaining user trust is therefore paramount. This requires a commitment from manufacturers and app developers to:
- Transparency: Clearly communicating how data is collected, used, and protected, as well as acknowledging the limitations and potential inaccuracies of the technology.
- Robust Security: Implementing strong security measures to safeguard user data.
- Verifiable Accuracy: Providing evidence of data accuracy through independent validation where possible.
- Explainability: Investing in XAI to make recommendations more understandable and trustworthy.
Market Snapshot: Key Players and Products Shaping the Future in 2025
The landscape of AI and wearable technology for running is dynamic and diverse, populated by established tech giants, specialized sports technology firms, innovative software startups, and traditional apparel companies embracing digital integration. Understanding the key players and product categories helps navigate this evolving market. Here are some of the best AI running apps and devices making waves:
Leading AI-Powered Running Apps
Running apps leveraging AI can be broadly categorized by their primary focus:
- Coaching / Training Plan Focused Apps: These apps are primarily designed to generate personalized and often adaptive training plans using AI algorithms. They analyze user data and goals to structure workouts leading up to a race or fitness objective.
- Examples: Runna (known for highly personalized plans targeting specific races), TrainAsONE (emphasizes adaptability and injury risk minimization), Humango (offers plans for multi-sport athletes), NXT RUN, RunBox (often highlights AI voice coaching), Coopah, RunMotion Coach, Run With Hal (digital version of Hal Higdon’s famous plans), Freeletics (broader fitness but includes running), Fitbod (focuses on strength but can integrate cardio).
- Gait Analysis Focused Apps: These specialize in analyzing running form, typically using smartphone video or dedicated sensors.
- Examples: Ochy (popular AI video analysis tool), Runeasi (professional-grade IMU sensor system for 3D analysis), OnForm (acquired Hudl Technique, used for video analysis across sports). Tools like Kinovea or Coach Logic might be used by coaches for manual or semi-automated analysis.
- Broad Fitness / Tracking Platforms with AI Elements: These are often large ecosystems that include run tracking alongside many other features, gradually incorporating AI insights.
- Examples: Strava (dominant social platform for runners/cyclists, introducing AI features like “Athlete Intelligence”), Garmin Connect (the companion app for Garmin devices, integrating features like Training Readiness, PacePro, and training plans), Apple Health / WorkoutDoors (Apple’s ecosystem integrates data, with third-party apps like WorkoutDoors offering advanced metrics), Nike Training Club / Nike Run Club (offers guided runs and plans, often free), Peloton App (includes running content).
- AI Workout Generators: A newer category using generative AI models to create training suggestions based on user prompts.
- Examples: While not dedicated running apps, tools like ChatGPT can generate basic plans if prompted correctly. Specialized strength AI like JuggernautAI indicates the trend towards generative AI in training plan creation.
Dominant Wearable Technology Companies for Runners
The hardware side of the equation is equally diverse:
- Major Watch / Tracker Players: These companies offer a wide range of wrist-worn devices and have strong brand recognition and large user bases.
- Examples: Apple (Apple Watch dominates the smartwatch market), Garmin (leader in dedicated GPS running and multisport watches), Fitbit (Google) (strong focus on general health, wellness, and accessibility), Samsung (Galaxy Watch line), Polar (long history in heart rate monitoring and sports science), Coros (gaining popularity, especially among trail and ultra runners for battery life), Suunto (known for robust outdoor watches). Companies like Huawei, Xiaomi, and Amazfit also offer competitive options, often at lower price points.
- Specialized Sensor / System Providers: These companies focus on niche hardware or systems, often targeting specific metrics or elite users.
- Examples: Catapult (GPS and physiological monitoring systems for professional teams), Whoop (subscription-based strap focused purely on recovery, strain, and sleep monitoring via HRV), Runscribe (advanced foot-mounted IMU sensors for detailed biomechanics), Stryd (pioneering foot pod for running power measurement), Nurvv Run (smart insoles for pressure and gait analysis), Sensoria (smart garments like socks), Kinexon (player tracking and analytics for team sports).
- Apparel / Shoe Companies (with Tech Integration): Traditional running brands are increasingly integrating technology or acquiring tech companies.
- Examples: Nike (Nike Run Club app integration, past FuelBand efforts), Adidas (partnerships and app integration), Under Armour (owns MyFitnessPal, previously had connected shoes), Asics, New Balance, Hoka, On Running, Brooks, Saucony (primarily footwear focused but part of the broader ecosystem runners engage with).
Here’s a summary of some key players and their focus within the AI and Wearable Technology for Running space:
Company/App Name | Relevant Products/Services | Key AI/Wearable Features | Market Focus/Notes |
---|---|---|---|
Apple | Apple Watch, Apple Health | GPS, OHR, EKG, Fall Detection, App Store Ecosystem | Dominant Smartwatch Player, Broad Ecosystem |
Garmin | Forerunner Series, Fenix, Connect App | Advanced GPS, OHR, HRV Status, Training Load/Readiness, VO2 Max, PacePro, Power Data | Leader in Running/Multisport Watches, Data-Rich |
Fitbit (Google) | Sense, Versa, Charge, Fitbit App | OHR, Sleep Tracking, Activity Monitoring, Readiness Score, EKG, EDA Scan | Health & Wellness Focus, Accessible Trackers |
Runna | Runna App | AI Personalized & Adaptive Running Plans, Watch Integration | AI Coaching Specialist, Race-Goal Oriented |
TrainAsONE | TrainAsONE App | AI Adaptive Plans, Injury Risk Minimization, Multi-Goal Support | AI Coaching Specialist, Data-Driven, Flexible Planning |
Ochy | Ochy App | AI Video-Based Gait Analysis, Biomechanical Metrics, Exercise Suggestions | Gait Analysis Focus, Accessible Biomechanics Assessment |
Runeasi | Runeasi Sensor & Software | IMU Sensor 3D Gait Analysis, Dynamic Stability, Impact Loading | Professional Gait Analysis, Physio/Performance Focus |
Whoop | Whoop Strap & App | Continuous HRV, Sleep, Strain & Recovery Monitoring | Recovery & Physiological Monitoring Focus, Subscription |
Strava | Strava App | GPS Tracking, Segments, Social Network, Route Discovery, Athlete Intelligence (AI) | Large Running/Cycling Community, Social Motivation |
Nurvv Run | Nurvv Smart Insoles & App | 32 Pressure Sensors, Cadence, Pronation, GCT, Pace Coach, Running Health Score | Insole-Based Biomechanics, Performance Optimization |
Sensoria | Sensoria Smart Socks & App | Textile Sensors, Foot Landing, Cadence, Real-Time Audio Coaching (Mara) | Smart Garment Focus, Form Correction |
Stryd | Stryd Foot Pod & App | Running Power Meter (Wind Adjusted), Pace, Distance, Running Dynamics | Running Power Specialist, Performance Metrics Focus |
Market Dynamics: Fragmentation, Specialization, and Integration
The current market is characterized by:
- Fragmentation: Numerous players operate in different niches, from hardware giants to specialized software startups.
- Specialization: Companies often focus on specific areas – Garmin on GPS watches, Whoop on recovery, Ochy on video gait analysis, Runna on AI coaching.
- Ecosystems: Larger players like Apple, Google (Fitbit), and Garmin aim to create integrated ecosystems where their devices and software work seamlessly together.
- Integration Needs: This fragmentation and specialization mean that runners seeking comprehensive insights often need to combine solutions from different providers (e.g., using a Garmin watch, a Stryd pod, and a separate AI coaching app). This makes interoperability and data sharing between platforms a critical consideration. Can your chosen app easily import data from your specific watch or sensor?
- Innovation Driver: While potentially confusing for consumers, this competitive landscape drives rapid innovation in sensor technology, AI algorithms, and application features.
The Road Ahead: Future Trends and Predictions in AI and Wearable Technology for Running
The field of AI and wearable technology for running is evolving at lightning speed. Innovation continues unabated, promising even more sophisticated tools and personalized experiences for runners in the coming years. Here are some key trends and predictions shaping the future:
Evolution of AI Algorithms: Smarter, Faster, More Personal
The intelligence powering these systems is constantly getting sharper:
- On-Device AI (Edge AI): A significant trend is moving AI processing from the cloud directly onto the wearable device itself. This offers several advantages:
- Faster Real-Time Feedback: Reduced latency means quicker responses for coaching cues or alerts.
- Enhanced Privacy: Sensitive data can be processed locally without necessarily being sent to the cloud.
- Reduced Network Dependency: Functionality is less reliant on a constant internet connection. This requires developing highly efficient AI models that can run effectively on the limited processing power and battery capacity of wearable devices.
- Generative AI (GenAI): We’re starting to see GenAI applied in fitness. Future applications could include:
- Hyper-Personalized Content: Generating unique watch faces based on performance data, creating novel workout variations, or even composing personalized motivational messages.
- Natural Language Interaction: More sophisticated AI coaches or chatbots that runners can interact with using natural language to ask questions about their training, data, or even nutrition.
- More Sophisticated Models: Research continues into more advanced ML and DL algorithms. Techniques like deep reinforcement learning (where AI learns through trial and error), transformer models (excelling at sequence data), and more complex neural network architectures promise:
- More Accurate Predictions: Improved forecasting of performance, fatigue, and injury risk.
- More Nuanced Insights: Ability to understand even more subtle patterns and complex interactions within the runner’s data.
- Better Handling of Uncertainty: Algorithms that can better quantify the confidence level of their own predictions.
Advancements in Wearable Sensors and Hardware: Better Data, Better Form Factors
The hardware powering intelligent running experiences is rapidly evolving, becoming both smaller and more potent. This evolution paves the way for increasingly integrated and insightful data capture.
We are witnessing the emergence of entirely new sensor modalities. Imagine wearables using miniature ultrasound to offer insights into muscle activation or deeper physiological states. Chemical sensors, perhaps integrated into patches or fabrics, could soon analyze biomarkers like lactate or hydration directly from sweat in real-time.
While these cutting-edge sensors develop, existing technologies are also seeing significant refinement. GPS tracking benefits from multi-band systems and better error correction, improving accuracy in tricky environments. Optical heart rate sensors are becoming more reliable across diverse skin tones and during intense activity. The IMUs crucial for wearable sensors for running analysis are capturing subtler movement nuances with greater sensitivity.
This quest for superior data aligns with hardware design progress. Miniaturization allows powerful sensors to fit into discreet forms like intelligent fabrics, skin patches, or smart rings. Battery life, a persistent challenge, is steadily improving, enabling longer tracking and more complex on-device tasks.
Crucially, on-device processing power is increasing. This allows more complex AI analyses to occur locally (edge AI), promising faster feedback, reduced latency, enhanced privacy, and better offline functionality.
Deeper Integration with Emerging Technologies: Connecting the Dots
The future isn’t just about individual device improvement; it’s about creating a richer, connected running ecosystem through integration with other technologies.
Augmented Reality (AR) could revolutionize the runner’s immediate perception. Smart glasses might overlay real-time data (pace, heart rate) directly in view, eliminating watch glances. AR could visualize virtual pacers or project navigation cues onto the path.
Virtual Reality (VR) offers powerful tools for indoor training, technique analysis, and rehabilitation. Runners could use VR for immersive simulations, virtual race courses, detailed biomechanical feedback, or AI-guided rehab exercises.
Integration with the wider Internet of Things (IoT) promises a more holistic health view. Wearable data could merge with information from smart home devices (sleep quality from mattresses), environmental sensors (air quality, temperature impacting training), or connected gym equipment, providing AI with richer context.
Towards Hyper-Personalization and Advanced Prediction: The N=1 Future
The ultimate goal, driven by sensor advancements and integration, is true hyper-personalization – training and recovery tailored precisely to the individual.
Future AI models will likely incorporate a much wider range of data inputs. Genetic information might reveal predispositions and training responses. Real-time mental state assessment could influence training intensity. Detailed nutrition logs could be correlated with energy levels. For women, menstrual cycle data will enable more nuanced training adjustments.
As AI digests this multi-dimensional data, its predictive capabilities will sharpen. Expect more accurate performance forecasts, earlier fatigue detection, and significantly enhanced AI for running injury prevention by identifying subtle risk patterns. Some even foresee wearables aiding in early illness detection by spotting baseline physiological deviations.
Market Growth Outlook: A Booming Sector
The increasing capabilities and adoption of these technologies fuel a rapidly expanding market. Research consistently forecasts strong, sustained growth for wearables, fitness trackers, GPS watches, health sensors, and the overall sports tech sector.
This growth is driven by rising health consciousness, continuous tech breakthroughs (AI, sensors), increasing adoption rates, and strong consumer demand for personalization. Regions like Asia-Pacific are emerging as particularly fast-growing markets, indicating a global trend.
The Expanding Data Ecosystem and the Central Role of AI
The foundation of future advancements is an ever-expanding data ecosystem, with AI acting as the essential intelligence layer. Data will increasingly come from sources beyond the wearable itself – environmental sensors, genetic information, nutrition logs, subjective runner feedback, and smart environments.
Creating this rich data tapestry enables hyper-personalization and advanced prediction. However, making sense of this vast, complex data requires sophisticated AI. AI algorithms are the key enablers for processing diverse datasets, identifying patterns, and delivering personalized plans, coaching, predictions, and integrated experiences.
Yet, AI development itself remains a critical factor. Progress hinges on creating AI that is robust, accurate, efficient (especially for on-device use), and explainable. Overcoming current challenges in accuracy, bias, explainability (the “black box” problem), and efficiency is vital to realizing the full potential of intelligent running technology.
Conclusion: Synthesizing the Present and Future of Intelligent Running
In summary, the integration of AI and Wearable Technology for Running marks a fundamental shift in athletic training and self-understanding. We’ve transitioned from basic tracking to an era of intelligent analysis, personalized guidance, and proactive health management.
The core synergy is clear: wearables provide the data, and AI provides the insights. This combination yields tangible benefits like tailored adaptive training, real-time feedback, detailed gait analysis, and promising avenues for AI for running injury prevention. These tools empower runners to optimize performance, boost motivation, and train more safely.
However, unlocking this technology’s full potential requires addressing significant challenges. Issues surrounding data accuracy, algorithmic bias, user privacy, cost and accessibility, technical limitations, and the risk of over-reliance need careful consideration and mitigation. Building and maintaining user trust through transparency, security, and proven efficacy is paramount.
The future trajectory points towards even smarter sensors, more sophisticated and efficient AI (including on-device and generative models), deeper integration with technologies like AR/VR and IoT, and ultimately, hyper-personalized running experiences unique to each individual. The market’s strong growth confirms the momentum behind this evolution.
Ultimately, the most successful application of this technology will remain human-centric. AI and wearables should augment runner intuition, coaching expertise, and the personal experience of running, not replace them. Prioritizing ethical considerations and user trust will be essential for responsible innovation. When this balance is achieved, AI and wearable technology can truly help runners of all levels maximize their potential and deepen their enjoyment of the sport.
This comprehensive exploration into AI and Wearable Technology for Running would not have been possible without the foundational work of numerous researchers, engineers, reviewers, and platforms who are actively shaping and documenting this exciting field.
We extend our sincere gratitude and respect for their dedicated efforts and invaluable contributions, which have significantly informed this article. For readers wishing to delve deeper into specific examples or research repositories mentioned, we encourage exploring resources such as Sensoria Fitness, NURVV Run, the extensive archives of PubMed Central (PMC), and publications from platforms like MDPI.
❓Frequently Asked Questions: AI & Wearable Technology for Running (2025)
🤖What is “AI” in running apps and wearables?
📈How does AI improve my training?
⏱️Which metrics can AI analyze from my runs?
🏆What’s the best AI running app in 2025?
🔋Do wearables drain quickly with AI features?
🛡️Is my data safe? Who owns my running information?
📱Can I use AI coaching without a smartwatch?
💸Are AI running wearables worth the investment?
🎯Can AI prevent running injuries?
🧬How accurate are these devices?
👟What’s the best device for gait analysis?
🌎Are AI wearables suitable for trail or ultrarunning?
💡Will AI “replace” human coaches?
🛠️Can I use multiple wearables together?
🚀What new features can I expect in late 2025?
⚡Are there free AI running apps?
👤Is AI training suitable for beginners?
🎽Can AI analyze my race performance?
🧑🔬What about scientific validation?
🔗Where can I learn more?
🏃♂️ Quick Quiz: How Well Do You Know AI & Running Tech?
- What metric is most commonly used to measure recovery in AI wearables?
Steps
Heart Rate Variability (HRV)
Calories
Shoe size - Which company is famous for their smart insoles providing real-time gait analysis?
Stryd
Garmin
NURVV Run
Apple - What is one main advantage of AI training plans compared to static PDF plans?
Lower price
Adapts to your progress in real time
No device needed
Works only offline - True or False: All wearables are equally accurate for heart rate variability (HRV) data.
True
False - Which feature is expected in late 2025 AI running tech?
Emotional state tracking
Carbon plate detection
Shoe size prediction only
No new features
✅ Quiz Answers
- 1: Heart Rate Variability (HRV)
- 2: NURVV Run
- 3: Adapts to your progress in real time
- 4: False
- 5: Emotional state tracking
🔗 Share this Guide
📚 Further Reading & Resources
How smart apparel is transforming running biomechanics. Best GPS Watches for Ultra Training
In-depth guide to the latest GPS devices for endurance athletes. What is Overtraining Syndrome?
How AI and wearables can help detect and avoid overtraining. Downhill Running Technique
Protect your legs using data-driven running form tips.
- Over 45% of recreational runners use AI-driven running apps or wearables. (Running Industry Association, 2025)
- Global running wearables & AI market exceeds $6.2 billion USD (MarketWatch, Q2 2025)
- Wearables with advanced HRV and recovery tracking increase training adherence by 37%. (Sports Tech Journal, March 2025)
- Peer-reviewed: “Artificial Intelligence in Endurance Sport” (2024)
🚀 Final Thoughts: The Future of Running Starts Now
Artificial intelligence and wearable technology are no longer futuristic buzzwords—they’re revolutionizing the way we train, race, and recover, right now. Whether you’re a data-loving veteran or just lacing up for your first 5K, today’s AI-powered tools can unlock insights and motivation you never knew you needed.
The real power comes when you combine smart tech with smart habits: listen to your body, stay curious, and use data as a tool—not as a limit. Every runner’s journey is unique, and the best technology is the one that helps you enjoy the run and reach your next goal.
- 🏅 Experiment with different wearables and apps—find what matches your style and goals.
- 🔍 Dive deeper into the science of running with our guides, FAQs, and expert tips.
- 🙌 Share your journey with the community—your story might inspire someone else!
Curious about the latest devices, research, or training strategies? Browse our latest blog posts or check the Further Reading section above for more resources.
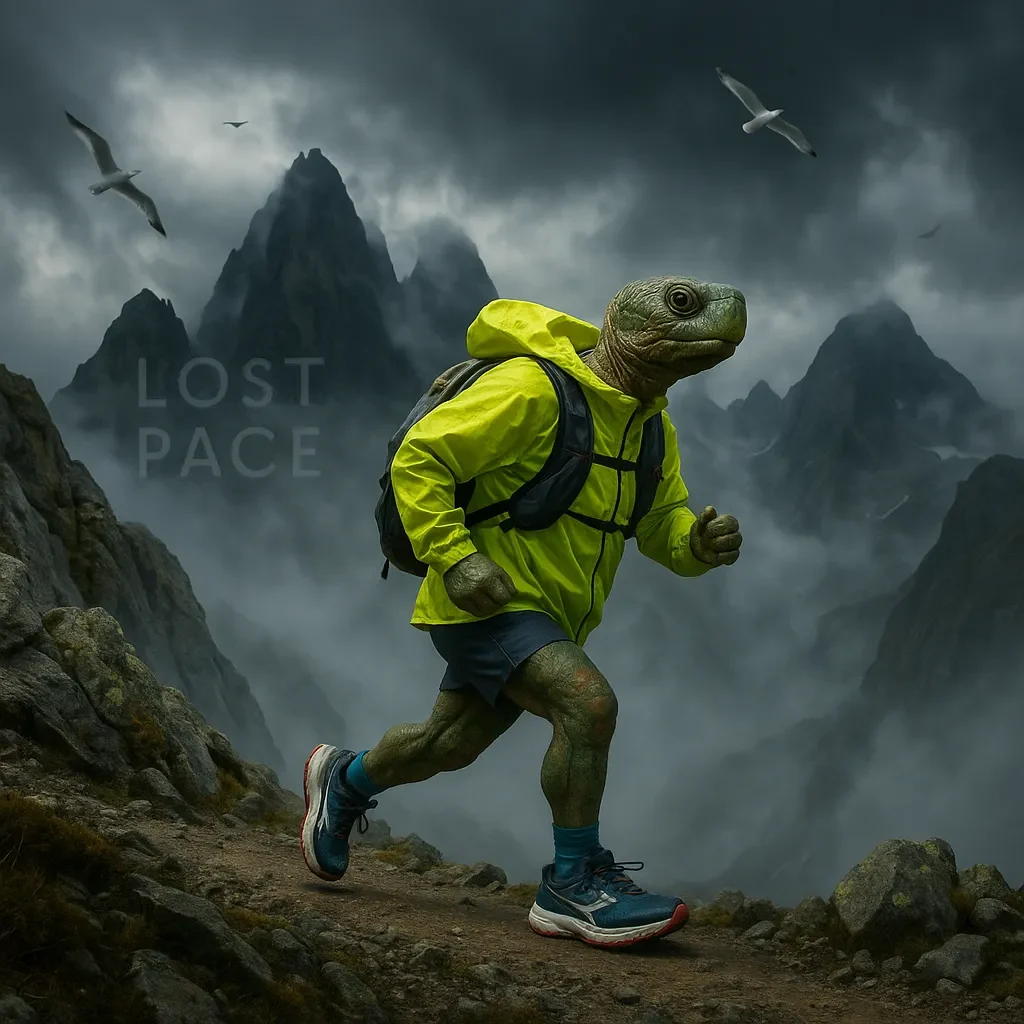
About the Author
Lost Pace is an ultramarathon runner, shoe-tester and the founder of umit.net. Based year-round in Türkiye’s rugged Kaçkar Mountains, he has logged 10,000 + km of technical trail running and completed multiple 50 K–100 K ultras.
Blending mountain grit with data, Lost analyses power (CP 300 W), HRV and nutrition to craft evidence-backed training plans. He has co-written 260 + long-form guides on footwear science, recovery and endurance nutrition, and is a regular beta-tester of AI-driven coaching tools.
When he isn’t chasing PRs or testing midsoles, you’ll find him sharing peer-reviewed research in plain English to help runners train smarter, stay healthier and finish stronger.
Ultrarunner · Data geek · Vegan athlete